Developer Insights
Join millions of viewers! Our engineers craft human-written articles solving real-world problems weekly. Enjoy fresh technical content and numerous interviews featuring modern web advancements with industry leaders and open-source authors.
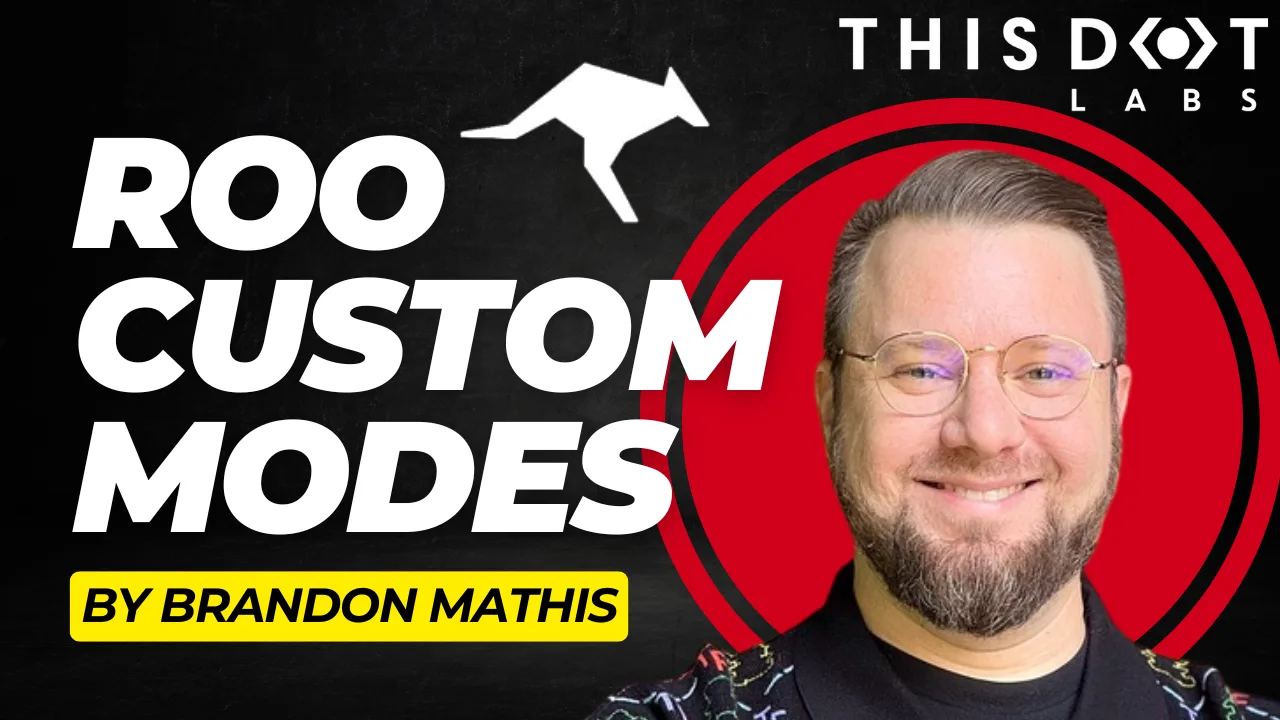
Roo Custom Modes
Roo Code is an extension for VS Code that provides agentic-style AI code editing functionality. You can configure Roo to use any LLM model and version you want by providing API keys....
Jun 13, 2025
6 mins
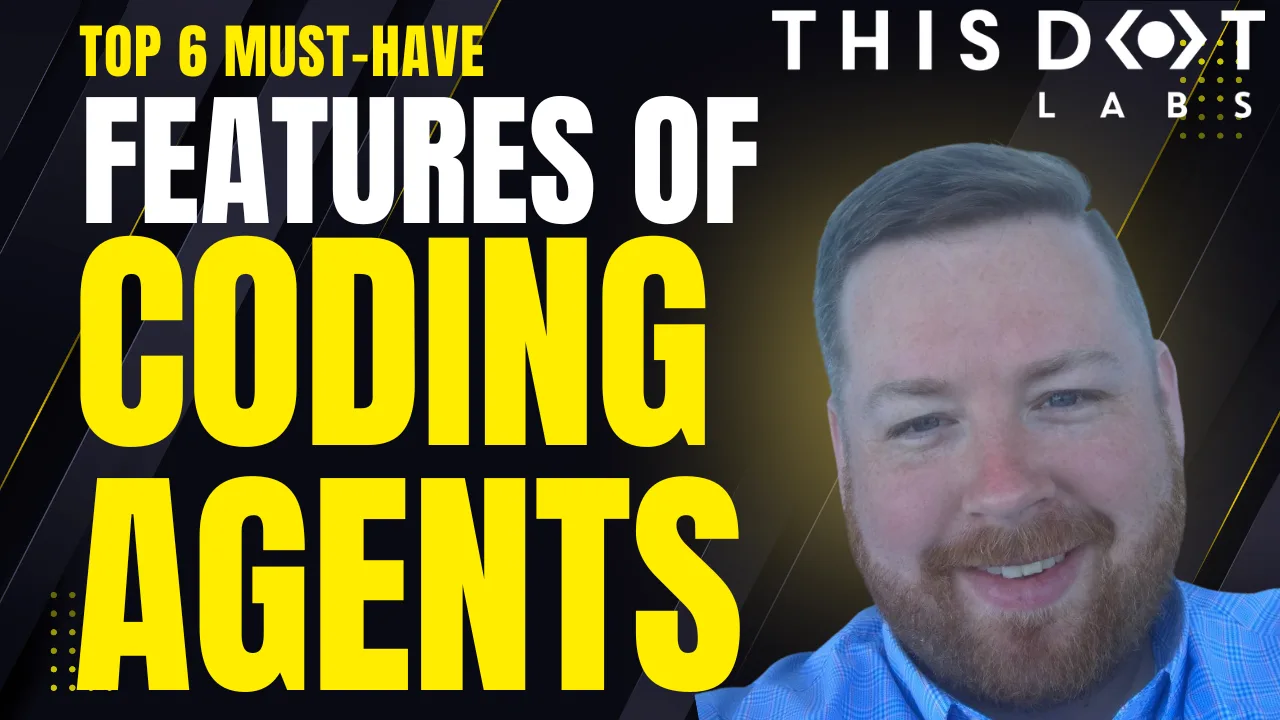
What Sets the Best Autonomous Coding Agents Apart?
Autonomous coding agents are no longer experimental, they are becoming an integral part of modern development workflows, redefining how software is built and maintained....
Jun 3, 2025
4 mins
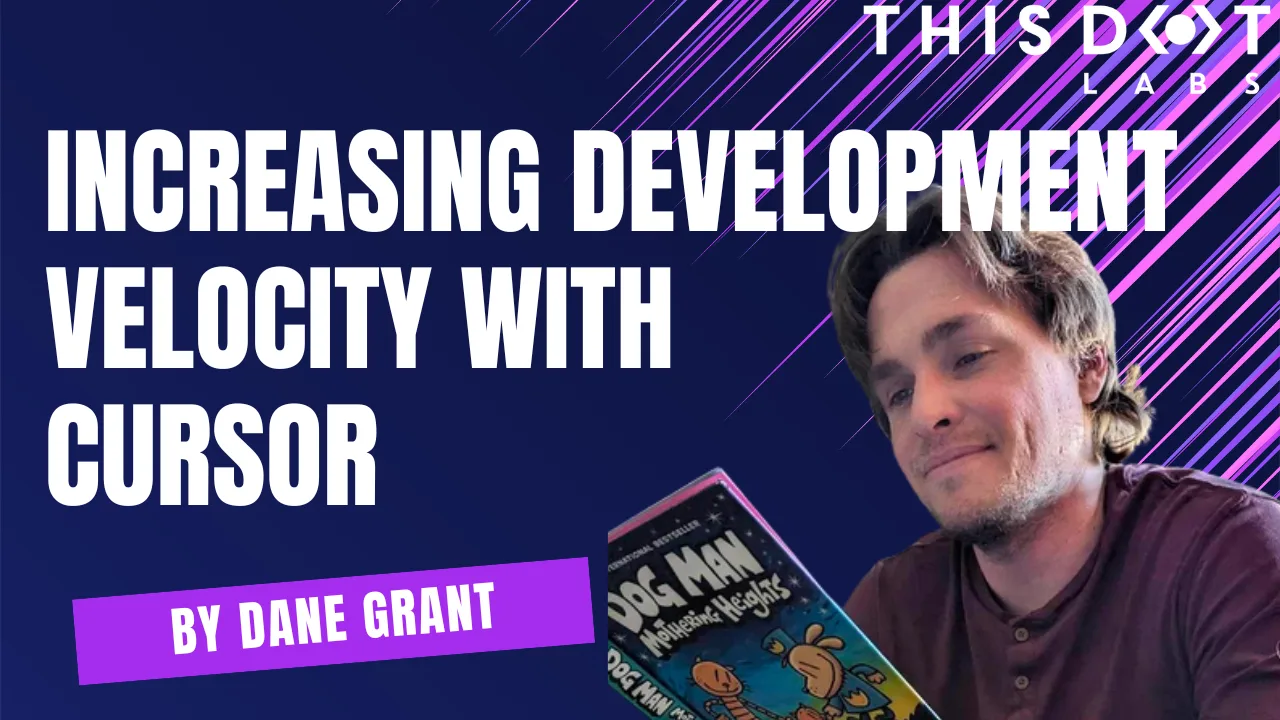
Increasing development velocity with Cursor
Boost your coding efficiency with Cursor, an AI-powered VSCode fork. Learn how features like Autocomplete, Ask, and Agent can streamline development, automate tasks, and enhance productivity....
Apr 18, 2025
11 mins
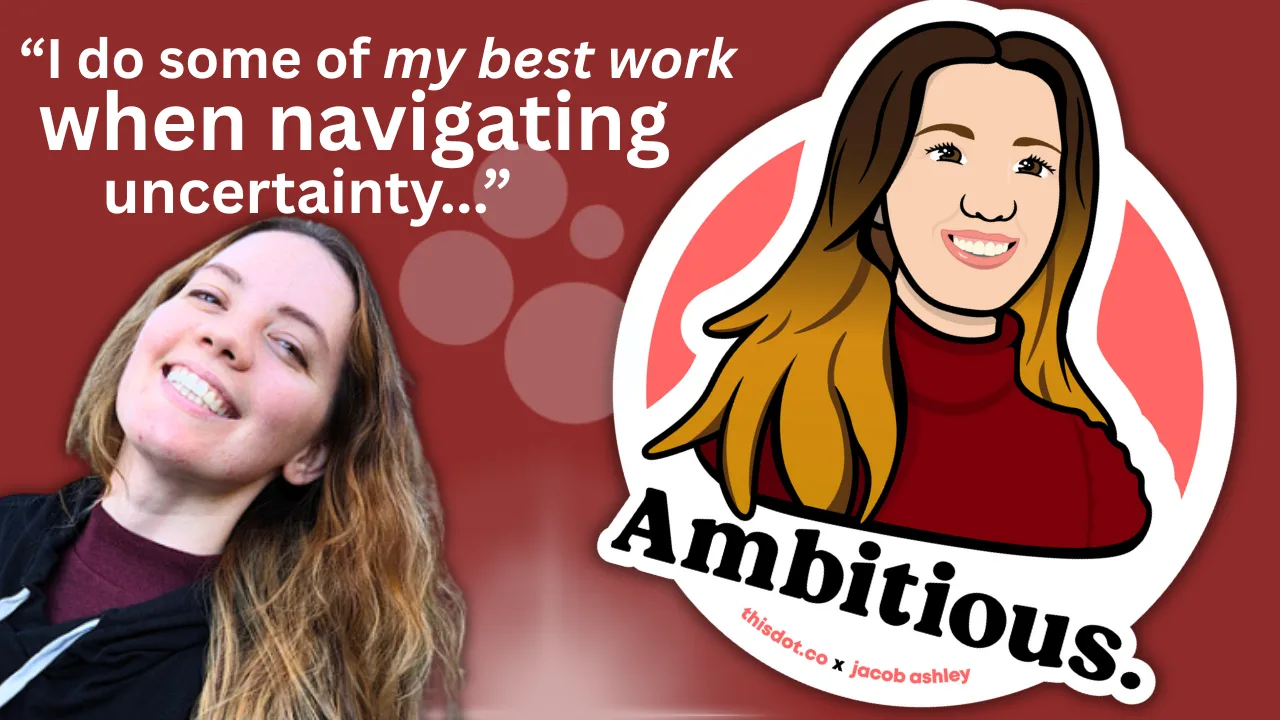
“Recognize leadership behavior early. Sometimes people don’t even realize it in themselves…” Kelly Vaughn on Product Leadership, Creating Pathways for Women in Tech, & Conferences
Some leaders build products. Some lead engineering teams. Kelly Vaughn is doing both. As Director of Engineering at Spot AI—a company building video intelligence software—Kelly recently expanded her role to oversee both Product and Engineering for their VMS offering. That shift means juggling strategy, execution, and team development, all while helping others step confidently into leadership themselves. And yes, she still finds time to speak at conferences and answer DMs from people navigating the same transitions she once did. We spoke with Kelly about spotting leadership potential early, why ambiguity doesn’t have to feel chaotic, and the lesson she learned the hard way about managing up. Stepping into Product Leadership Kelly’s new title might look like a promotion on paper, but the shift is more philosophical than anything. > “Engineering leadership is about execution,” she says. “Product leadership is about defining why we’re building something in the first place.” Now leading Product and Engineering for Spot AI’s VMS product, she’s talking to customers, researching market trends, and making smart bets on where to invest next. It’s a role she’s clearly energized by. > “I’m really looking forward to dedicating time to shaping our product’s future.” Thriving in Ambiguity Some people panic when problems are fuzzy or undefined. Others use it as fuel. > “There are two key traits I see in people who handle ambiguity well,” Kelly says. “They stay calm under stress, and they know how to form a hypothesis from a vague problem statement.” That means asking the right questions, taking action quickly, and being totally okay with pivoting when something doesn’t pan out. It’s no surprise that these same traits overlap with great product thinking—a mindset she’s now leaning into more than ever. > “I do some of my best work when navigating uncertainty,” she adds. Read Kelly’s blog on embracing ambiguity in Product! Creating Leadership Pathways for Women in Tech When asked how leaders can create more leadership pathways for women in software engineering, Kelly stressed that it is not a passive process. > “Senior leaders need to be proactive,” Kelly says. “That starts with identifying and addressing bias across hiring, promotions, and day-to-day interactions.” She emphasizes psychological safety—so women feel confident advocating for themselves and others. But she also knows not everyone feels ready to raise their hand. > “Don’t wait for someone to ask for a title change or a growth opportunity. Recognize leadership behavior early. Sometimes people don’t even realize it in themselves yet.” On Stage, In Real Life Kelly’s no stranger to the tech conference circuit—often giving talks on engineering leadership and team growth. Her biggest source of inspiration? Conversations with people trying to make the leap into leadership. > “I might use the same slide deck at three conferences,” she says, “but the talk itself will be different every time.” Rather than sticking to a script, she likes to share recent examples from her own work, tailoring the delivery to the audience in front of her. It keeps things relevant, grounded, and never too polished. Between setting product strategy, mentoring the next generation of leaders, and hopping from one tech conference to the next, Kelly Vaughn is showing what it means to lead with clarity—even when things are unclear. She’s not here to tell you it’s easy. But she will tell you it’s worth it. Connect with Kelly Vaughn on Bluesky. Sign up for Kelly Vaughn’s Newsletter! Sticker Illustration by Jacob Ashley....
Apr 4, 2025
3 mins
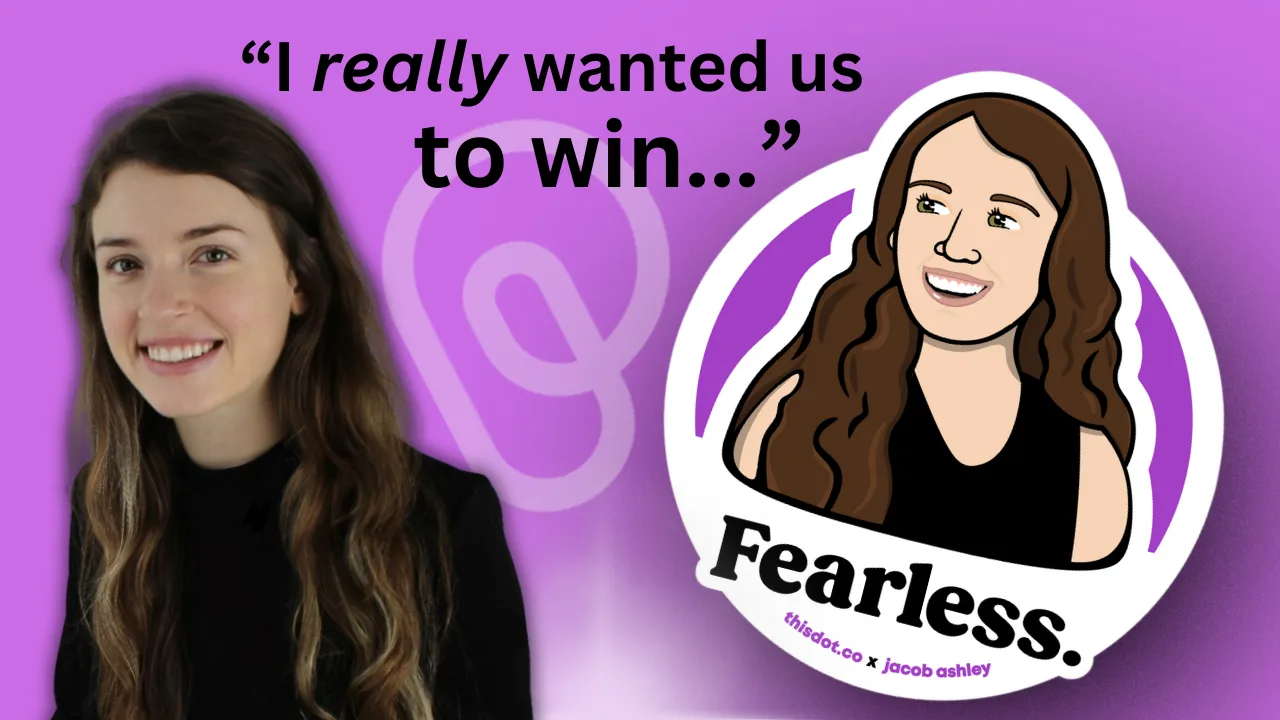
“It Sounds a Little Dystopian, But Also Kind of Amazing”: Conversations on Long Term AI Agents and "Winning" Product Hunt with Ellie Zubrowski
Ellie Zubrowski doesn’t walk a traditional path. In the three years since graduating from a university program in Business Administration, she biked across the U.S., studied Kung Fu in China, learned Mandarin just for fun, and completed the #100DaysOfCode challenge after deciding she wanted a career switch. That same sense of curiosity and willingness to jump into the unknown now fuels her work as a Developer Advocate at Pieces, where she leads product launches, mentors job seekers, and helps developers learn how to best leverage Pieces’ Long-Term Memory Agent. Her journey into tech was guided not just by a want to learn how to code and break into the industry, but by a fascination with the structure of language itself. > “There are so many parallels between human languages and programming languages,” she says. “That realization really made me fall in love with software.” > We spoke with Ellie about launching a #1 Product Hunt release, her predictions for AI agents, and why conferences don’t have to break your budget. Launching LTM-2 to the Top of Product Hunt Recently, Ellie led the launch of Pieces’ Long-Term Memory Agent (LTM-2), which took the top spot on Product Hunt—a major win for the team and their community. > “I’m super competitive,” she admits. “So I really wanted us to win.” The launch was fully organic—no paid promotions, just coordinated team efforts, a well-prepared content pipeline, and an ambassador program that brought in authentic engagement across X, Discord, and Reddit. She documented their entire strategy in this blog post, and credits the success not just to good planning but to a passionate developer community that believed in the product. Following a successful performance at Product Hunt, Ellie is committed to keeping Pieces’ user community engaged and contributing to its technological ecosystem. > “Although I’m still fairly new to DevRel (coming up on a year at Pieces!), I think success comes down to a few things: developer adoption and retention, user feedback, community engagement, and maintaining communication with engineering.” Why AI Agents Are the Next Big Thing Ellie sees a major shift on the horizon: AI that doesn’t wait for a prompt. > “The biggest trend of 2025 seems to be AI agents,” she explains, “or AI that acts proactively instead of reactively.” Until now, most of us have had to tell AI exactly what to do—whether that’s drafting emails, debugging code, or generating images. But Ellie imagines a near future where AI tools act more like intelligent teammates than assistants—running locally, deeply personalized, and working in the background to handle the repetitive stuff. > “Imagine something that knows how you work and quietly handles your busy work while you focus on the creative parts,” she says. “It sounds a little dystopian, but also kind of amazing.” Whether we hit that level of autonomy in 2025 or (likely) have to wait until 2026, she believes the move toward agentic AI is inevitable—and it’s changing how developers think about productivity, ownership, and trust. You can read more of Ellie’s 2025 LLM predictions here! The Secret to Free Conferences (and Winning the GitHub Claw Machine) Ellie will be the first to tell you: attending a tech conference can be a total game-changer. “Attending my first tech conference completely changed my career trajectory,” she says. “It honestly changed my life.” And the best part? You might not even need to pay for a ticket. > “Most conferences offer scholarship tickets,” Ellie explains. “And if you’re active in dev communities, there are always giveaways. You just have to know where to look.” In her early days of job hunting, Ellie made it to multiple conferences for free (minus travel and lodging)—which she recommends to anyone trying to break into tech. Also, she lives for conference swag. One of her all-time favorite moments? Winning a GitHub Octocat from the claw machine at RenderATL. > “She’s one of my prized possessions,” Ellie laughs. Proof here. 🐙 Her advice: if you’re even a little curious about going to a conference—go. Show up. Say hi to someone new. You never know what connection might shape your next step. Ellie’s Journeys Away from her Desk Earlier this year, Ellie took a break from product launches and developer events to visit China for Chinese New Year with her boyfriend’s family—and turned the trip into a mix of sightseeing, food adventures, and a personal mission: document every cat she met. (You can follow the full feline thread here 🐱) The trip took them through Beijing, Nanjing, Taiyuan, Yuci, Zhùmǎdiàn, and Yangzhou, where they explored palaces, museums, and even soaked in a hot spring once reserved for emperors. > “Fancy, right?” Ellie jokes. But the real highlight? The food. > “China has some of the best food in the world,” she says. “And lucky for me, my boyfriend’s dad is an amazing cook—every meal felt like a five-star experience.” What’s Next? With a YouTube series on the way, thousands of developers reached through her workshops, and an eye on the next generation of AI tooling, Ellie Zubrowski is loving her experience as a developer advocate. Follow @elliezub on X to stay in the loop on her work, travels, tech experiments, and the occasional Octocat sighting. She’s building in public, cheering on other devs, and always down to share what she’s learning along the way. Learn more about Pieces, the long-term LLM agent. Sticker Illustration by Jacob Ashley...
Mar 28, 2025
4 mins

“ChatGPT knows me pretty well… but it drew me as a white man with a man bun.” – Angie Jones on AI Bias, DevRel, and Block’s new open source AI agent “goose”
Angie Jones, VP of Developer Relations at Block, champions developer advocacy, AI ethics, and leadership. She’s leading discussions on AI governance, bias in career tools, and *goose*, an open-source AI assistant for developers....
Mar 13, 2025
4 mins
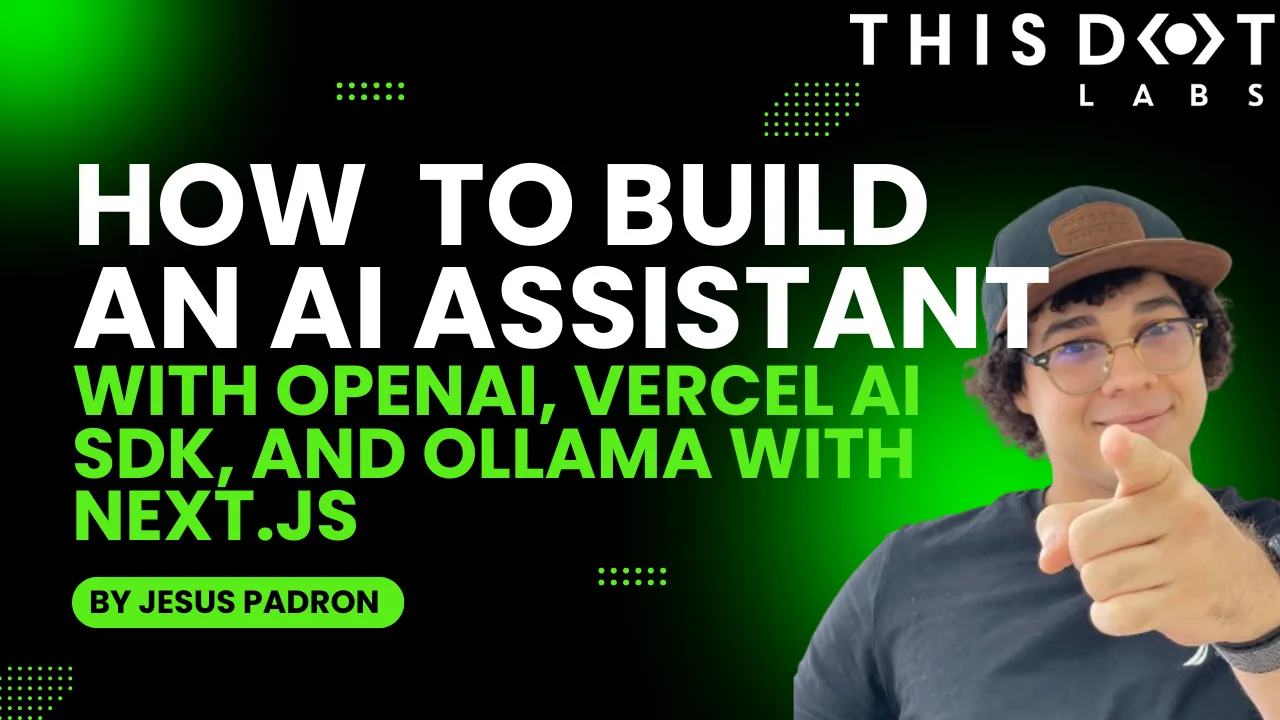
How to build an AI assistant with OpenAI, Vercel AI SDK, and Ollama with Next.js
Learn how to run Llama 3.1 locally in a Next.js app, send audio to it, and play responses back to users....
Sep 27, 2024
8 mins
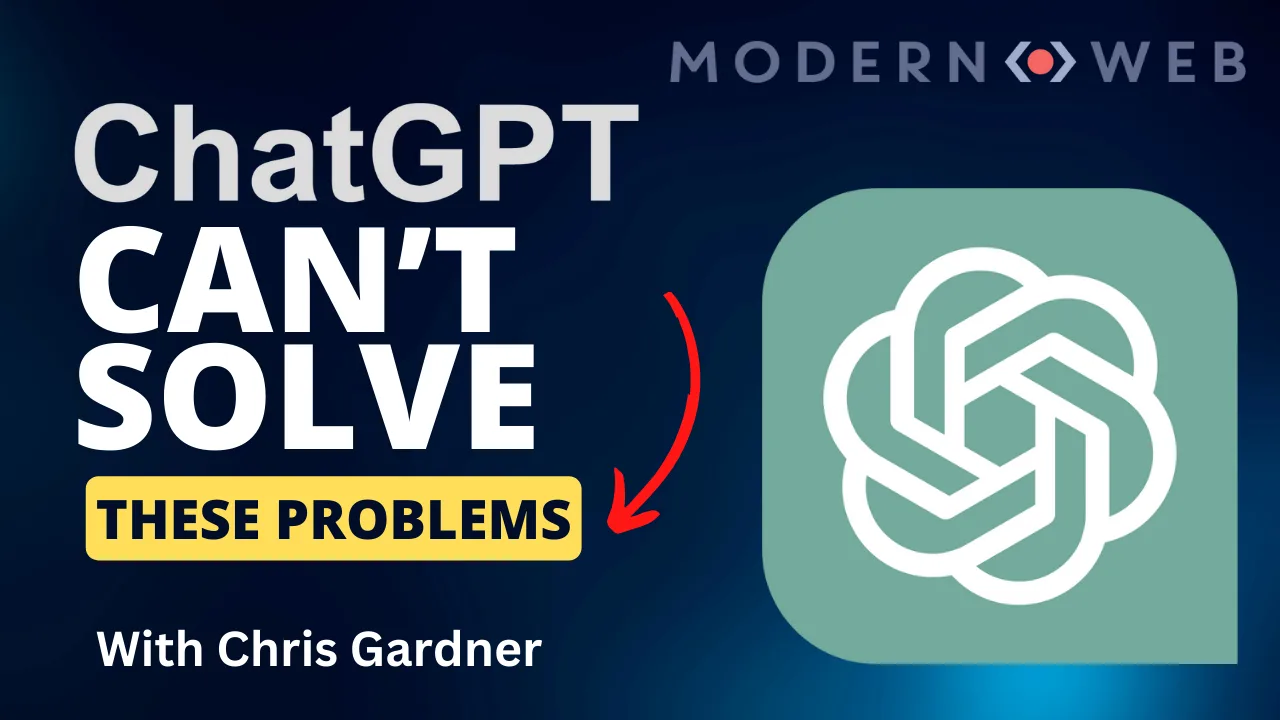
ChatGPT can't solve these problems! with Chris Gardner
Chris Gardner talks neural networks, covering fundamental concepts, historical development, and practical applications. It’s important to understand the difference between artificial intelligence (AI) and machine learning, and the role of neural networks in solving intricate problems with vast datasets. This conversation centers around the intricacies of training neural networks, particularly as they become more complex with multiple layers. Chris and Rob touch on the fascinating yet daunting nature of neural networks, discussing their ability to learn beyond human comprehension. Turning to the practical side of using neural networks, Chris shares the existence of libraries that exist to simplify the process of building a network, enabling users to input data, specify parameters, and entrust the system with the training. Both caution about the biases inherent in the data and the responsibility associated with working on machine learning models. They address challenges related to ethics, highlighting the difficulties in identifying biases and emphasizing the delicate balance between excitement and caution in the evolving field of machine learning. Listen to the podcast here: https://modernweb.podbean.com/e/chris-gardner/...
Jan 16, 2024
1 min
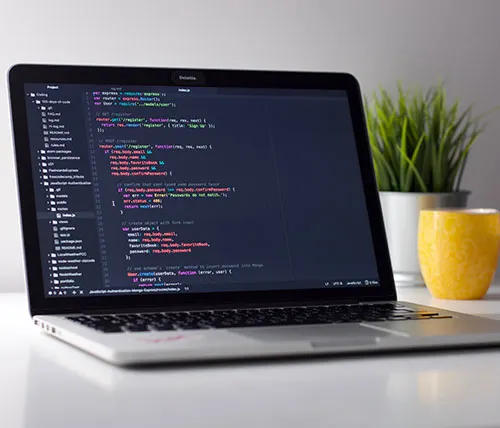
Let Purpose Drive Your Artificial Intelligence Transformation
From administrative and analytics tasks present in nearly every industry, to niche processes that serve only a handful of businesses, the diversity of how we apply Artificial Intelligence grows, seemingly by the moment. And with it, the size of the market is exploding. In fact, less than half a decade ago, this market was valued at $644 million, and half a decade from now, it is expected to be worth nearly $118 billion. When discussing the growth of AI, many have a hard time separating it from the advancement of AI. While the latter will naturally follow the former, the vast majority of companies that are integrating, and expanding the size of this market, will be in a constant state of catching up to some of the world’s foremost leaders in AI advancement. And I’m here to tell you, that’s okay. Because, as the growth of the AI market continues, the diversity in humans that will use it, and human demand that will be met by it, will grow too. And your responsibility as an executive or leader is not necessarily to push the envelope of AI, or discover the next game changing function at which the world can marvel; it is to meet the needs of the humans, be they your employees or customers, who will benefit from your integration. Of course, this is a very exciting time in the history of advanced digital technologies. Despite how far we have come, when we consider the potentially unending future of this transformative technology, we realize that AI is still in its infancy. As such, we often approach from a performance advancement mindset. However, if you confine your definition of performance to objective technical metrics, you may find yourself perpetually pursuing faster load times, smaller bundle sizes, and more impressive features. This may work out for your team if you have an unending line of investors, or get incredibly lucky. But even some of the biggest companies that neglected to first think of their user experience above all else have found themselves victims of Icarian pitfalls that have shelved their projects, depleted their financial resources, and denied users of products that they need. I do want to note, however, that the focus of this article isn’t to suggest that we shouldn’t strive to advance our understanding of the “nuts and bolts” of AI and other transformative digital technologies. But it’s time to make tough decisions about your company’s place in the AI marketplace. Do you have the budget, the demand, or a responsibility that requires you to implement the most cutting edge technologies available to the market? The answer for most companies is, no. For many, their future with AI is a continual, and incremental process of meeting the minimal needs of their customers, employees, or processes. And I am here to say that not only is this okay, but it’s a good thing. In this article, I implore you to look at AI as a tool for promoting human excellence and experience, and rethink what it means for AI technology to be “performant”. Ultimately, I want you to feel inspired and empowered to begin your digital transformation voyage by placing people and purpose at the helm. RETHINKING PERFORMANCE According to leading educational non-profit Autism Speaks, 1 in every 59 children born today will fall somewhere on the autism spectrum. Although symptoms are diverse, many individuals with this diagnosis struggle with normative social conventions in a manner that impacts their daily lives. Due to advancements in our understanding of autism, it’s now typically diagnosed in early childhood, tasking many parents with the responsibility of helping their children navigate a world that better accommodates neurotypical behaviors. Laura Krieger is one of these parents. Matthew, her eight year old son, has autism, and struggles to read others’ emotions. The pair were featured in a PBS Newshour segment on Brain Power, a company founded by award winning neuroscientist and entrepreneur, Ned Sahin. Brain Power’s product is a Google Glass aided software that utilizes both AI and augmented reality technologies to help kids with learning differences build skills such as identifying emotions, and maintaining eye contact, through play. When Krieger plays one of these skill building games with her son, she can’t help but break out in tears, saying she feels like he can truly see her for the first time. Krieger is not responding to just how low the latency of the codebase powering the software is, or whether its deploying the latest architectural concepts. She likely doesn’t care whether the software was created using Amazon Web Services, TensorFlow, or a proprietary system. What she does care about is getting the chance to connect with her son in a way she never thought possible. And when we look at Brain Power through the eyes of children who use it, we see its strength in its mode of education. Rather than running users through drills or lesson plans, it rewards them through collaboration, natural interaction, and a gamified points system. When you see the children using the system, they aren’t marveling at the natural feel of the interface that betrays its complexity. They’re simply having fun. This is the type of performance for which we should strive. THE CASE OF WATSON FOR ONCOLOGY In 2013, IBM launched a partner project with The University of Texas MD Anderson Cancer Center to create a “Watson for Oncology” software that would help doctors identify and prescribe courses of action for cancer treatment. After pumping $62 million dollars into the project, MD Anderson officially shelved it in 2017, halting their pursuit of a cure for cancer. But the problem wasn’t a technical one. That’s to say, it was not found within the codebase. It was with the data being processed by it. The reason that MD Anderson pulled the plug on the project, which would eventually be revealed by StatNews after reviewing internal slide decks from MD Anderson, was that the program was prescribing “unsafe and incorrect” treatment plans to real patients after the product had been sold to hospitals around the world. Anderson sourced the problem back to IBM engineers and New York City-based Memorial Sloan Kettering Cancer Center, who were responsible for training Watson for Oncology. It was later discovered that their ML training process relied on a relatively small collection of hypothetical oncological cases rather than using actual patient data. Of course, we cannot presuppose intent. I am of the opinion that IBM and Kettering truly wanted to provide a product that would revolutionize oncology treatment, and save lives. But it is hard to imagine a reason why a company creating a product meant to help doctors treat their patients in the field, would not have trained their AI software with data produced by doctors working with actual cancer patients. So after indefinitely stopping this project, MD Anderson had exchanged $62 million, and four years of its time, to create a product that may have reflected some of the most advanced technical concepts available at that time, but is completely useless, in its current form, for the purpose it was meant to serve. Imagine where we could be, how many lives could have been saved or prolonged, and how much money would have been saved, in the nearly three years since this product was pulled, if developers had placed equal focus on their users and the purpose of their product as they did the technical elements. MEETING NEEDS *“As researchers, we make decisions about what our AI systems can do. It may not necessarily be optimal. It may not be necessarily efficient if you look at all of the metrics… but it may be optimal with respect to the human… which means that the system works.”* - Ayanna Howard, Ph.D Chair, School of Interactive Computing Georgia Institute of Technology Kaden Bowen of Lincoln, Nebraska shares his father’s passion for cars. Though he is non-verbal due to cerebral palsy, he asks his father, James, to “go for a ride”, multiple times a day with the help of a digital talkboard. James dreamed of taking his son on a roadtrip in a vehicle that was more stylish and fun than their wheelchair-accessible van. He searched high and low for a sporty car that he could modify to at least allow him to store a foldable wheelchair, until he realized that the hatchback of a standard Corvette might just be big enough for one. Two weeks later, the duo took a 728 mile round trip to the Corvette museum in Bowling Green, Kentucky in their brand-new (to them) Corvette, which came standard with a trunk big enough for Kaden’s chair. The Bowens don’t seem to over-complicate the accommodations that they have put in place for their son. To help increase his level of independence, they outfitted their home with Amazon Echo devices that are sensitive enough to understand the commands that are programmed into Kaden’s talkboard. Using a combination of the two technologies, Kaden can do a lot on his own. He can call his parents on the phone, stream videos on Netflix, and operate lights. It seems so obvious, but it really is quite inventive. Sure, one day we will have widely accessible neural link technology that will allow Kaden to circumvent the talkboard, and do so much more than what is permitted by an Echo. But just like his dad’s Corvette, that comes standard with enough storage space, sometimes, the best fixes are the best fixes because they are available, and they work. The technologies that Kaden uses may not boast the best metrics, feature the most jaw dropping functions, or offer the most direct route to helping him achieve increased independence. I’m sure that the communication between the talkboard and the Echo device is not always perfect. But the combination of these two relatively affordable, and accessible technologies have given Kaden more autonomy with reliable, usable services, and that, in and of itself, *is* high performance. AI INITIATIVES ARE FAILING These past few years have proven to be extremely exciting for AI technologies, and businesses are responsive, with a 2019 Gartner report showing that 37% of the nation’s leading enterprises are or are shortly planning to integrate some form of AI into their products or processes. This percentage reflects a 270% growth in that statistic when compared to research conducted in 2015. And this proportion bumps up to 62% when we look at Supply Chain and Logistics, and reaches nearly 80% when discussing the Healthcare industry. But these awe-inspiring stats come with troubling predictions that, through 2020, roughly 80% of enterprise AI programs will remain in a limbotic state of development due to their inability to properly scale with their organizations. In essence, we may be creating fabulous algorithms, with wildly impressive features and functions that may become little more than multi-million dollar proof-of-concepts. And this might be okay for some businesses who have the resources to pursue multiple avenues and digital transformation. But I am of the belief that most companies that have yet to enter AI space by now will depend on a significant ROI from the programs that they start over the next few years. For these companies, it is imperative not only to balance resources, and create realistic time-frames for integrating and/or shipping their products, but to stay diligent against the propensity for AI programs to become isolated within an organization. With all of the buzz around AI, and the seemingly endless supply of jaw dropping products and services coming out of the world’s foremost information technology companies, it’s natural that smaller programs want to keep up with the Joneses. It is, therefore, the responsibility of executives, and others in business development roles, to remain involved with their AI development programs to ensure that products and services do not outpace the demands and capacities of their customers or businesses. WORKING SYSTEMS *“The current wave of Artificial Intelligence is going to hit a peak inside of the enterprise. But when it does, it’s not going to be a monumental revolution of technology, but rather a monumental revolution of people.”* - Traci Gusher Partner, US Leader- Artificial Intelligence Analytics and Engineering, KPMG There is a lot of chatter about the need for enterprises to integrate AI powered technologies into their workflows, and products. Anxiety about the need to introduce this transformative technology is warranted. Products like Alexa, Siri, and Echo Dot are changing consumer expectations, while Forrestor predicts that, in 2020, 25% of Fortune 500 companies will include AI building blocks in their automated processes. From product to process, AI is infiltrating nearly every sect of business. That being said, it is a mistake for companies to rush an AI program without first internalizing and developing concepts of how the resulting products will better the lives or capacities of those who will use it. I get it. It’s so tempting to want to patent the next game changing system or algorithm, but if your customers, users, or employees, could be equally, if not better served by a simpler system, or another company’s proprietary tools, what is the point? Is it 100% necessary that your business be on the cutting edge of Artificial Intelligence? Will it truly better your products, services, workflows, or customers? AI has such an amazing capacity to enrich the human experience, be that personal, or professional. We overemphasize minute technical details without giving that same attention to the aspects of user needs, and experience at our own peril. Don’t feel the need to push the boundaries of our understanding of transformative technologies simply to implement your own products and services. All you need is something that sees your user, empowers them, strengthens their skills, and most importantly, works. Ready to begin your digital transformation journey, but don’t know how to start? Don’t hesitate to reach out to the team at This Dot Labs by emailing hi@thisdot.co....
Feb 21, 2020
10 mins
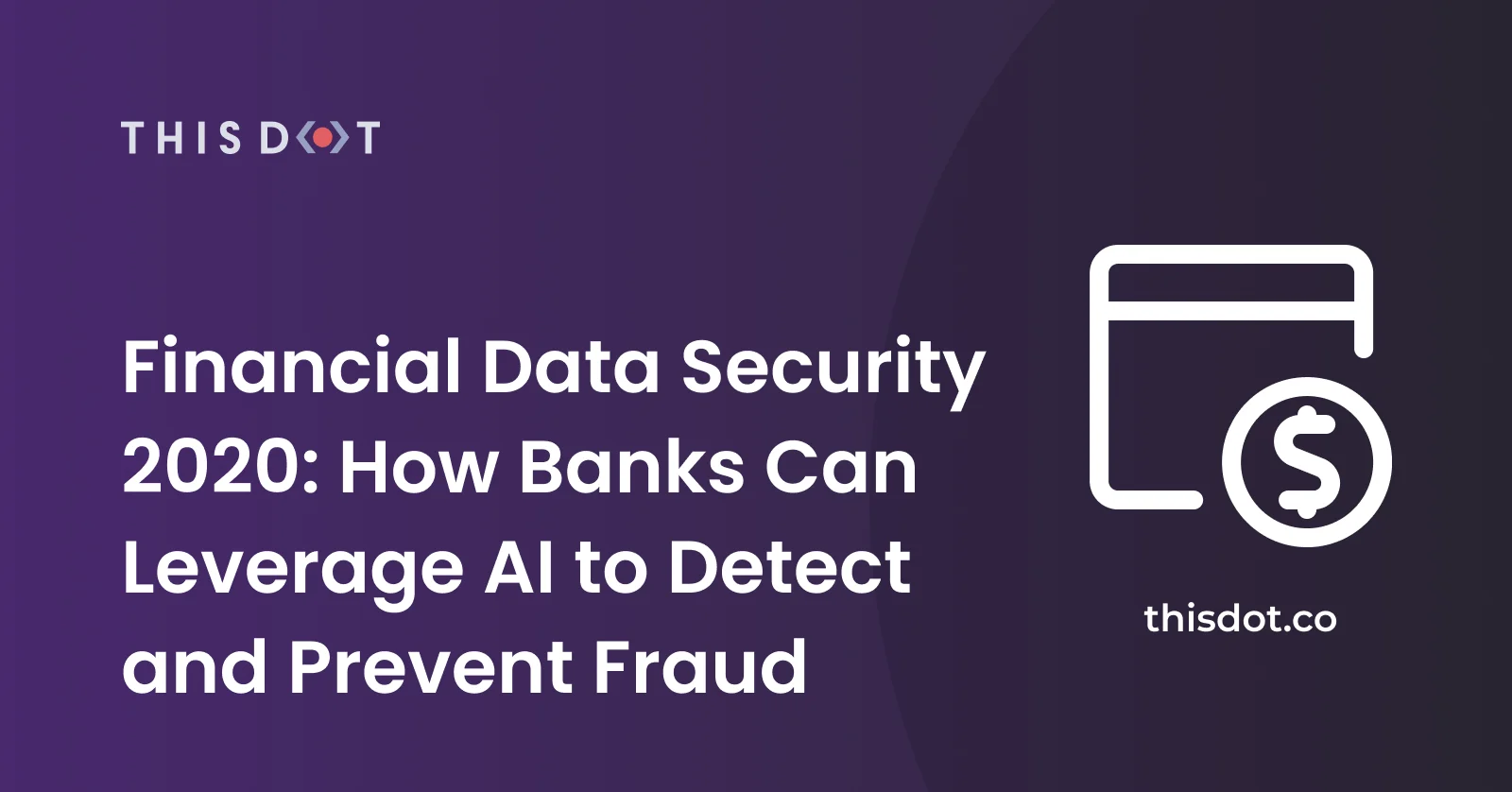
Financial Data Security 2020: How Banks Can Leverage AI to Detect and Prevent Fraud
As the volume of data grows and becomes more integral to daily and long term enterprise operations, the need to secure that data, and identify potential threats to it has never been more important. Cybercrimes are on the rise, with supply chain attacks up 79%, and reports of malicious PowerShell scripts increasing 1000%, in 2019 alone. A snapshot of 2018 fraud statistics presented by the FTC shows that the total identified fraud losses for individual Americans was about $1.48 billion, with the median loss per reportant equaling $375. And these statistics only reflect a portion of the 1.4 million total known instances of fraud, 75% of which did not result in any financial loss. For institutions, fraud threats can be financially devastating, resulting in losses due to theft, misrepresented financial reports, settlement costs and legal fees, operational disruption, and damaged customer and business relationships. Fraudsters are leveraging powerful, and widely available tech tools to mine businesses and individuals for important data with malicious intent, and it is incumbent that financial institutions apply the most advanced tech tools for combatting this. Promoting Customer Security with AI Most financial institutions use rule-based algorithms for alerting and blocking the potentially fraudulent transactions within all customer accounts. While this method has long been a sufficient mode of identifying fraudulent spending, the advancement of the digital age, and the expansion of mobile banking, means that individuals are keeping their money in a growingly diverse number of places, and routinely make transactions all over the world from single locations. This change has made it difficult for companies to employ one-size-fits-all predictive models to secure all customer accounts, since the diversity in user profiles and legitimate financial behaviors has outgrown them. Artificial Intelligence, however, is making it possible for financial institutions to create dynamic user profiles that track the behavior of individual account holders, and tailor fraud markers to their unique spending patterns. Kount, one company that has deployed its own proprietary AI model, for example, has found that their payments fraud detection accuracy has doubled when compared to predictive models, all the while maintaining a response rate of less than half a second. Implementing a successful AI fraud detection solution not only increases the volume of accurate detection, but decreases the instances of false positives, saving financial institutions the time and resources expended on human fraud analysis, falsely frozen accounts, and the need to constantly update predictive models. Protecting Data in the New Digital Age In late 2019, RiskedBased Security released a mid year report claiming that 2019 was on track to be the worst year for breach activity, with over 4.1 billion records compromised in the first 6 months. When we look at the total number of exposed records that same year, 61.7% belonged to financial institutions, including American Express, Suntrust, Capital One, Discover, and Lincoln Financial. Although falling victim to only 6.5% of all reported security breaches, financial companies tend to be most devastated by attacks due to the volume, variance, and sensitivity of their records when compared to that of other businesses. In the advanced digital age, data is not only crucial to protect for the interest of your customers, but also to prevent the distribution and corruption of information used to propel crucial operational decisions. Companies do not only have the responsibility to protect their customers against identity theft and fraudulent spending, but must also promote their own interests by safeguarding their most valuable digital assets. Financial institutions are massive, and as newer technologies are introduced alongside more antique, but nonetheless useful software and hardware, the challenge of protecting sensitive information at scale grows with every passing day. And just as our technologies are diversifying, so too are the threats against it. By implementing AI technologies, companies no longer need to develop seemingly endless and rapidly evolving solutions to potential security threats. Instead, companies can mobilize their existing data to create or implement intuitive softwares, able to identify real time security threats, and take appropriate action both with and without human intervention. How to Start Your AI Journey in 2020 According to IDC predictions, worldwide AI spending in 2019 was expected to be 44% higher when compared to data from the previous year. Further analysis also suggests that the banking sector is the second highest AI investor, with total spending believed to fall around $5.6 billion. With the emergence of blockchain technology threatening to dramatically revolutionize the banking sector, financial services companies will need to maintain their appeal to individuals and enterprises by leveraging their data to create more secure, performant products, services, and experiences. AI’s use in promoting data security is only one of the myriad applications of this transformative asset, expected to drive global trade operations as we enter a new era of advanced digital technologies. AI and Machine Learning are both tools that financial institutions must use to improve customer experiences, uncover novel business opportunities, and drive the direction of their future operations. The journey to full digital transformation is one of small, incremental steps. Financial services companies interested in AI integration do not have to start by creating full fledged, proprietary security systems, but can invest in future success by preparing their data, and implementing a simple AI based foundation for handling internal functions before ramping their technologies up to handle more critical aspects of their enterprise operations. Take this opportunity to set your company up for success as our digital capabilities grow both in capacity and necessity for modern global trade. By working with This Dot Labs, financial institutions learn more about how AI can support their unique operational needs, plan a pathway for its place in their future technical programs, and even start implementing some of the world’s most cutting edge technologies into your daily workflows....
Jan 29, 2020
5 mins
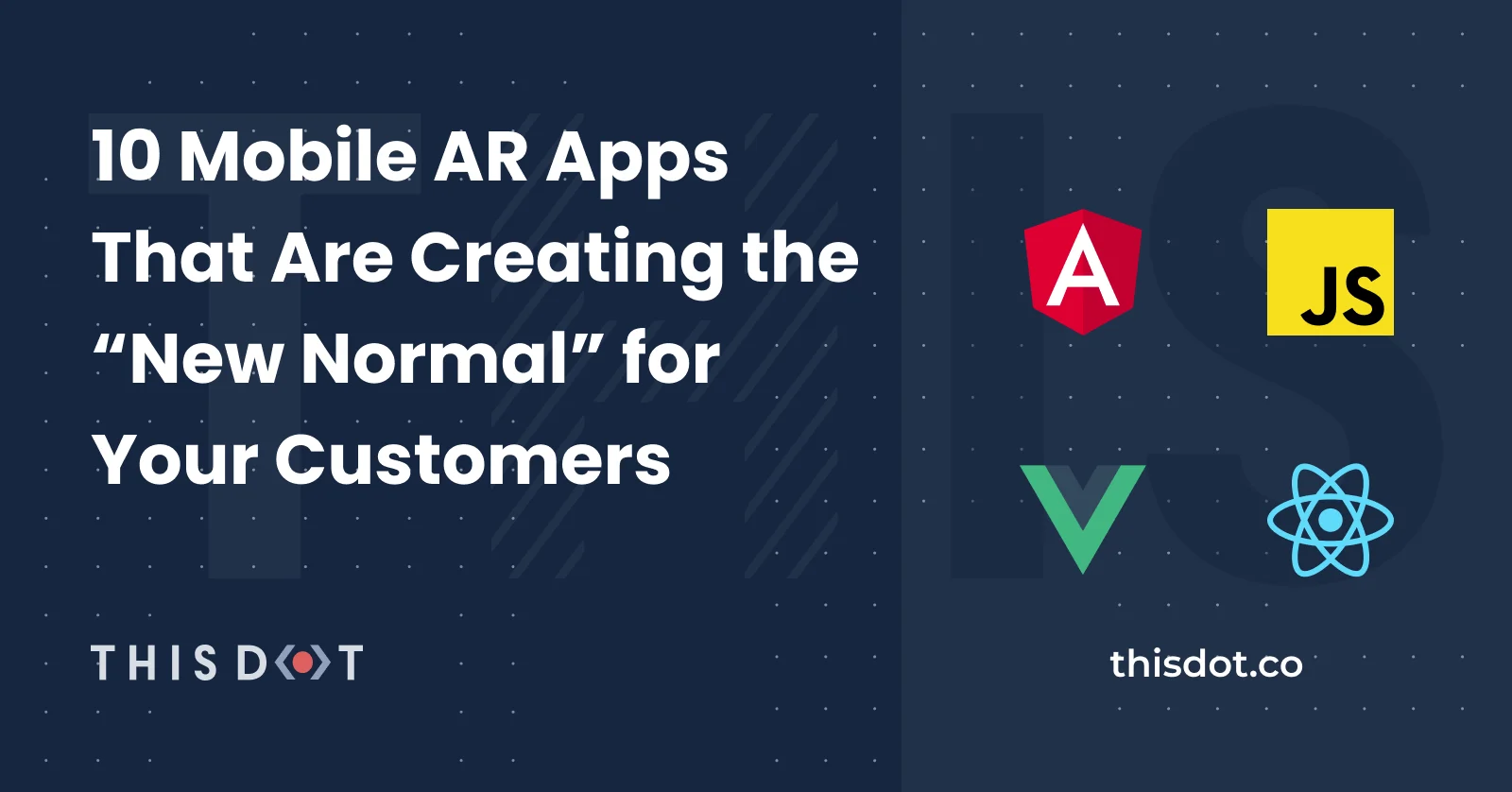
10 Mobile AR Apps That Are Creating the “New Normal” for Your Customers
Check out these 10 AR integrated mobile apps that are changing not only the way consumers interact with the physical world, but also their expectation for mobile app performance....
Jan 7, 2020
7 mins
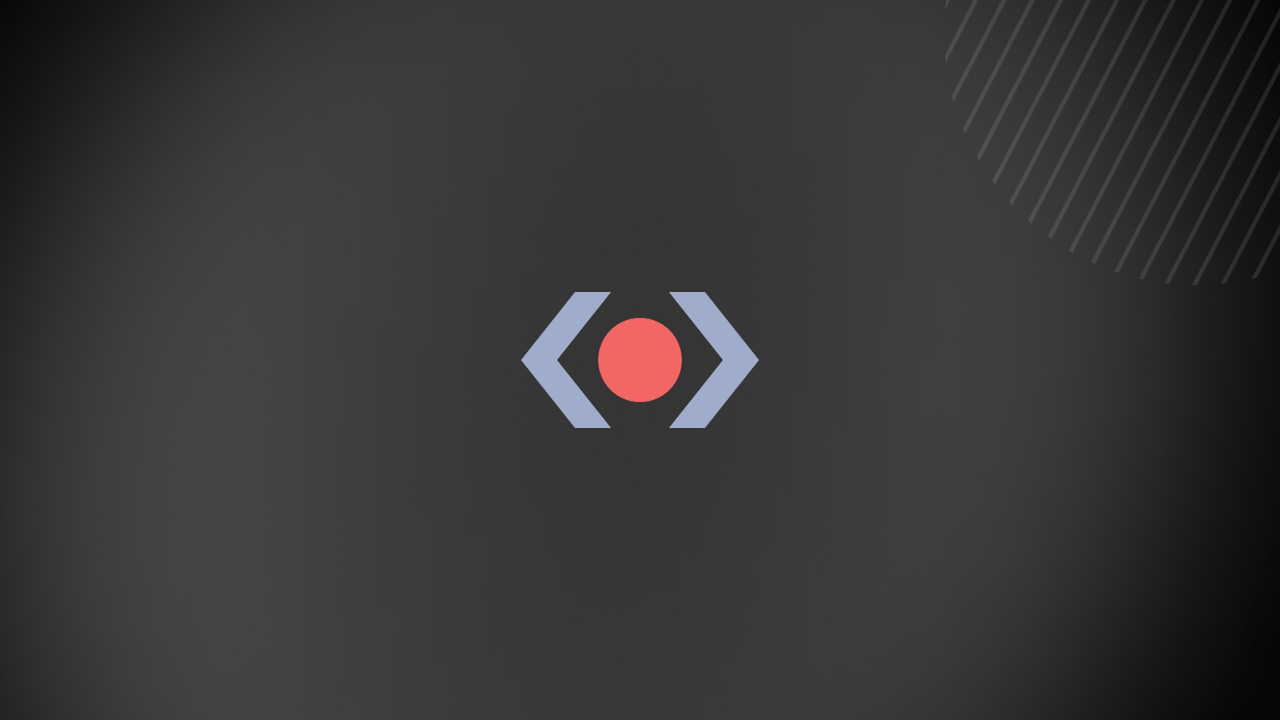
What We Can Learn From These 10 Companies That Are Implementing AI/ML in Unique and Exciting Ways
The Facts According to a 2019 Gartner survey of three thousand CIOs across a variety of industries, the number of enterprises that utilize AI technology has grown roughly 230% over the past four years. This is a particularly startling number for companies who have yet to integrate this transformative technology, since its growth has placed the estimated percentage of organizations that employ some form of AI at 37%. Some executives, leading companies within the other 63%, may brush these stats off, believing that they don’t stand to benefit enough from AI right now to justify investing in significant digital transformation. These companies, however, may find themselves playing catch up with competitors who have found creative ways to leverage this technology within their specific industries. Just take a look at these 10 trailblazing companies who are teaching us the value of modern technical innovation through the fascinating ways that they use AI! Blue River Tech: Shrinking Chemical Use in Agriculture It has been nearly 60 years since the publication of Rachel Carlson’s Silent Spring, which decried the indiscriminate use of pesticides and herbicides in commercial agriculture. Though we have seen different chemical and policy changes attempt to address the issues raised in the text, none may be as revolutionary as Blue River Tech’s AI driven farming equipment. Founded in 2011, the company has since been incorporated by leading agricultural machinery company, John Deere, in an effort to grow its flagship “See & Spray” product. This device uses deep learning algorithms, similar to those used in facial recognition software, to discern weeds from crops, and apply appropriate amounts of chemical herbicides. Not only does this decrease the need for farm managers to employ, and train multiple workers to spot and correctly treat invasive, and highly threatening plants, but it can, according to Business Development VP, Ben Chostner, “save up to 90% of the amount of herbicide that you would spray if you were spraying the entire field”. Cinalytic: Modeling the Financial Success of Big Budget Movies As the ways that audiences consume film advertisements continues to fragment by the day, the cost for marketing films-- one of the largest areas of investment for production companies-- is growing exponentially. However, this troubling news comes with a silver lining. According to 2018 data compiled by IBIS Worldwide, the compound annual growth rate for the American film industry is roughly 2%, which outpaces the nation’s overall economic growth. This saddles production companies with the task of finding new ways to optimize ROI at a time where there has never been so much money to make, but where it also requires such a financial investment to make a movie. This is where Cinalytics steps in. The LA based startup’s AI powered platform considers fifteen unique attributes that can predict the potential success of a film. Using historical data about the performance of thousands of films, the program is able to identify relationships between the financial success of films, and factors like casting, genre, and ratings, to forecast the revenue that producers might be able to expect from any given project. Users can then make changes, such as swapping out one actor for another, or changing the scale of the film’s release, to see how different pre-production decisions may impact their performance metrics. Not looking to remove the human element from filmmaking, CEO Tobias Queisser believes his company’s tool can “supplement the creative process”, and help producers think through artistic decisions with the added confidence provided by predictive data. Brain Power: Augmenting Reality for People on the Autism Spectrum According to leading educational non-profit Autism Speaks, 1 in every 59 children born today will fall somewhere on the autism spectrum. Although symptoms are diverse, many individuals with this diagnosis struggle with typical social conventions in a manner that impacts their daily lives. As autism is typically diagnosed in early childhood, many families are tasked with the responsibility of helping their children navigate a world that better accommodates neurotypical behaviors. Brain Power is using transformative technologies, including AI and AR (augmented reality) to provide autistic children, and adults, with learning experiences that help them with daily tasks and life skills. Having consulted a diverse array of families, healthcare providers, and counselors, and ran clinical trials, the company has created software that allows users to build skills such as identifying emotions based on facial cues, and maintaining eye contact. Utilizing Google Glass, the software’s zero UI interface reacts to the user’s eye motion while simultaneously reading their environment to detect not only the presence of other people, but also another person’s facial expressions. In one demo, featured on a PBS News Hour, Laura Krieger, the mother of an eight year old child with autism, plays a game with her son where she emotes surprise, which Brain Power’s software reads and then prompts her son to identify among two possible options. This, however, is just one of the wide array of skill building tools and games that Brain Power has delivered through its cutting edge software suite. Stanford Computational Policy Lab: Standardizing Judicial Practices Bias mitigation is a significant concern in modern policing. When many think about AI implementation in police and judicial processes, they shudder at the potential risks. Because AI/ML relies on prior data, some suggest that implementing AI software will increase bias given the historical over-policing of certain groups in America. Though they haven’t created a predictive software, Stanford’s Computational Policy Lab has pursued a unique way to combat racial prejudice in the judicial, and very well may be laying the groundwork for creating more equitable crime predicting AI in the future. Stanford’s Computational Policy Lab recently partnered with the San Francisco Police Department to develop a tool that strips arrest records of information that might evoke such conscious or unconscious biases when being presented to the District Attorney’s Office. The software uses name-entity recognition technology to identify and remove not only an arrestee’s race, but also descriptive factors that might allude to a suspect’s demographic information from unstructured text. These include physical descriptions like eye, and hair color, names, locations, and neighborhoods where the subject lives or was arrested. The software will also remove information alluding to the identity of involved officers, including names, and badge numbers to even further prevent the DA’s office from making unfair inferences. The goal of this is to reduce or eliminate the probability that racial bias might impact what charges are levied against a suspect. Though San Francisco commissioned the non-profit to develop this software, Stanford has delivered this software to the city at no charge, and intends to release the software widely for any city to use. IBM and McCormick & Company: Enhancing Human Creativity Remember in 2011, when IBM’s Watson annihilated champion contestants Ken Jennings and Brad Rutter on a special episode of Jeopardy? Well Watson has since stepped away from the podium and into the kitchen. Through a partnership with McCormick & Company, IBM has deployed “Chef Watson” to generate AI conceived spices that will be sold under the iconic seasoning and condiment company’s branding. The company hopes to eliminate the need for consulting often dozens of developers to create a product by leveraging their decades of recipes in order to invent standardized metrics for understanding flavor as data. This removes the issue of developer preference and bias by assigning objective metrics to ingredients that then can be combined to create highly original recipes that are less conventionally referential, and thus less easily replicated. This technology will not totally remove the human element of product development, but may lead to a 70% reduction in labor according to Hamed Faridi, the company’s Chief Science Officer. Since not all elements of the flavors can be easily and totally objectifiable, there still remains a need for human testing and augmentation. However, as McCormick believes, using IBM’s technology will allow even less experienced product developers to work as efficiently as developers with twenty years of experience. And the company believes its first AI generated product will be released by the end of 2019. Nauto: Uniting with Human Drivers to Create Safer Roads Though Stanford researchers have suggested that there will be 10 million self driving cars on the road by the year 2020, concerns still abound regarding the safety of full automated vehicles. Even though a McKinsey study has shown that 90% of driving related deaths could have been avoided had the drivers been using AI powered vehicles, there is something unnerving, for some, about placing their lives in the hands of a computer, and at this time, the technology is prohibitively expensive for most. And this concern multiplies for fleet companies, whose vehicles are both extremely expensive to own as it is, and capable of doing considerably more damage in an accident scenario. Nauto, the commercial fleet safety company, has created Prevent, an AI-powered device that employs deep learning algorithms to identify when drivers are distracted based upon their facial expressions, and eye lines, as well as their spatial relations to other cars, and whether they commit traffic violations. Then, the system is able to notify the driver, as well as the driver’s employer, in real time, about the nature of their risky behavior. However, CEO Stefan Heck stressed, in an interview with Tech Crunch, that the technology’s intention is, “to be really focused on keeping the driver safe without being intrusive… We want to help human drivers, not just rat them out to their boss.” As of the publication of this article, Nauto reports that it has detected nearly 49 million high risk driving events, and have saved commercial fleet companies an estimated 976 million dollars. Inspirata: Driving Precision Medicine For individuals who develop cancer, early detection greatly improves their chances of being able to successfully treat the illness both in the short and long-term. However, accurate and timely detection is not always easy when the signs that pathologists need to identify in order to diagnose and treat cancers consists of such a wide breadth of information. Inspirata, an oncological informatics company, is helping us realize a future where our doctors can utilize the pinpoint accuracy of artificial intelligence to pull critical data from not only our physical sample slides, but also our radiology narratives, as well as unstructured clinical text. These softwares rely on deep learning algorithms that collect user input and are able store that data in to make inferences about future input that it encounters. For example, if a pathologist identifies a cluster of cancerous cells in a sample slide and reports this finding to the software, the software can use that information to help future pathologists identify similar cases. This, of course, also relies on the software’s astounding capacity to map the structure of human cells and quantify that data in a manner that can be understood and reapplied in the future. Similarly, Inspirata has continued to expand its suite of workflow tools to include natural language processing that extracts data from millions of clinical texts deriving from a network of over 400 global healthcare providers. In this sense, doctors are not only able to benefit from the forward progression of medical technology, but this implementation of machine learning, in the words of Inspirata Founder and Executive VP, Dr. Mark Lloyd, “gives pathologists the power to not only contribute to precision medicine, but to drive precision medicine.” LeadGenius: Automating the B2B Sales Pipeline In modern sales, there are generally two broadly defined strategies: old school, reliant on cold calls, handshakes, and remembering the names of your clients’ kids, and new school, where representatives connect over Linkedin, and hook potential clients by pushing material relevant to their product or service. But is there something even newer than “new school”? Not too unlike the way that Inspirata generates and leverages data by relying on doctors to teach its AI technology to identify diagnostic data, LeadGenius benefits from the talent and know-how of over 500 researchers from 40 countries to train its software to do much of the legwork involved in sales. LeadGenius uses crawlers to search the web for potential clients, and is able to identify and isolate roughly thirty data points about a company, including its name, industry, revenue, and the technologies it uses, in order to determine the business’ suitability as a customer. Since much of this information is not contextualized or well structured, LeadGenius’ software must not only rely on language processing, but must also be trained by seasoned salespeople who know how to find, and identify this crucial data. The software is even capable of emailing prospective clients using natural language as if developed by a salesperson themselves. In a 2015 talk at a Silicon Valley Data Engineering Meetup, LeadGenius Co-Founder and Chief Scientist, Anand Kulkari made the startling claim that machines will replace salespeople in 10 years. But this doesn’t mean that salespeople will be out of work- what it means is that professionals will have to spend far less time searching out companies, analyzing their buying patterns, and initiating contact. This will allow representatives the ability to get in front of more potential customers and simply focus on closing. Solutions 4 Health: Tailoring Support for Cigarette Cessation According to the CDC, 68% of US smokers report wanting to quit. However, in the same year that these statistics were obtained, less than 8% of adult smokers reported being able to successfully give up smoking that same year. For many smokers, the support of tailored counseling and monitoring can be a significant motivator to maintain their cessation journey. That being said, lack of access to these services, which are often cost prohibitive or otherwise difficult to access, can leave many looking to lead healthier lives without any support. Leading health technology company, Solutions 4 Health, is on a mission to address such healthcare inequalities with its line of various AI powered innovations. One of these technologies is “Quit with Bella”, an iOS mobile application that allows users to text and even speak with an intelligent chatbot, who offers responsive, personalized support to help them quit smoking. The tech team behind the app utilized Microsoft’s JavaScript Object Notation (JSON) to transmit data via human-readable text. This allows users to freely speak with “Bella”, who can process their language input, and source helpful advice on a range of behavioral and product solutions from a vast databank. Unlike a conventional, human counselor, Bella is accessible at any time, day or night, and is undoubtedly any early iteration of the sort of life-like AI healthcare providers that the healthcare industry will utilize to better meet patients’ needs. Inturn: Strategizing Supply for Retailers Overstock is one of the most crippling sources of waste for millions of global retailers, with the current market creating a 170 billion dollar annual problem. Many, if not all, major retailers depend on being able to sell their overstock to discount and resale companies to reduce the loss in revue. However this can be a weeks long process for retailers, which often involves massive excel spreadsheets, and moving their inventory from showroom to showroom. The time and difficulty of this practice not only results in increased labor costs, and decreased human productivity, but also places companies under immense pressure to move their products before products and fashions become out of date. Inturn is leveraging the power of AI to help companies sell their overstock more quickly and strategically. Founded in 2013, the company offers a suite of different tools to automate what is often the impossible manual task of identifying which products are not selling at appropriate volumes, and expediting their liquidation to discount retailers. The software relies on analyzing large volumes of legacy data across multiple sources to cross reference the sales patterns of a retailer’s products based on multiple categorical factors such as size, color, style, fabric. Not only allowing companies to better strategize when they move inventory to the discount supply market, the software can also aggregate all of the information contained across multiple inventory databases, and present that information on a single platform for easy use by interested buyers. This allows companies to reach more potential resellers, secure better deals, and ultimately move their overstock more quickly, increasing margins by an average of 23%. The Future The purpose of this article is not just to highlight 10 companies that are utilizing AI to disrupt and advance their particular industries. The creative implementation of AI, Machine Learning, and other transformative technologies is on the rise, and with it, comes their demystification. The technological capabilities of AI are vast and have yet to be fully explored, however, our baseline understanding of its innumerable capacities, and the ways to unlock these functions is there. In putting together this list, we attempted to find companies who are each implementing digital transformation in unique ways. However, when you explore the technologies that power their products and services, you realize the concepts behind them aren’t all that dissimilar. Both Blue River Technologies, and Nauto have leveraged video-based object-recognition to address devastating problems unique to their industries. Solutions 4 Health, and the Stanford Computational Policy Lab operate in wholly unrelated spaces with drastically different goals. However, both are using machines to isolate, identify, and extract data from uncontextualized human speech and writing. Even Cinalytic, the film pre-production advisor, is utilizing very similar data analytics concepts that McCormick has employed to help them develop the next big food seasoning! If you or the company you work for has not yet investigated how AI and machine learning can help you better automate your workflow and services, while drastically reducing waste and maximizing profit, you are not alone. Remember that an estimated 63% of major enterprises have yet to successfully integrate digital transformation into their operations! That being said, the International Data Corporation (IDC) predicts that companies will spend roughly $1.25 trillion dollars by the end of 2019 on their transformative digital journeys. It is clear that major corporations are recognizing the long-term investment value for AI and ML integration. As these companies and organizations continue to invest, they are likely to see significant improvements not only in the quality of their products, services, and work processes, but will be setting themselves up for long-term economic growth that we can not possibly imagine at this time. Continued investment also gives these companies a great foundation off of which they can grow their technologies through further technical integration as new problems arise, and new capabilities are imaginable. Beginning your process of digital integration doesn’t have to start with a multi-million dollar overhaul of your technical stacks. It can start with small improvements to the functionality of your existing technologies, or creating simple software to better automate services you already provide. Where you do not want to find yourself, as a business, is being in the position where, in order to compete with companies in your own industry, you are forced to make significant investments over a short period of time, to meet the new standards created by these revolutionary technologies. There has never been a better time to start your journey. So many companies have done the legwork of not only creating the multiple, diverse, open source technologies that are leveraged to achieve these advancements, but they have also done the equally difficult work of imagining ways to effectively apply these technologies to challenges that impact their businesses and industries. As an unintegrated business, you have a unique opportunity to expand your technical program at a time where the science and concepts abound, but where the features offered by AI and ML are not yet the baseline standard for enterprises in the modern world. It now falls upon C-level executives to make the often difficult, but obvious choice to begin transforming their digital technologies now. The future of your business depends on it....
Oct 31, 2019
15 mins
Let's innovate together!
We're ready to be your trusted technical partners in your digital innovation journey.
Whether it's modernization or custom software solutions, our team of experts can guide you through best practices and how to build scalable, performant software that lasts.