Adding React to your ASP.NET MVC web app
In this article, we'll take a look how and why you might want to choose React to handle your front end considerations, and let ASP.NET manage the backend
Architecture
First, let's consider the range of responsibilities that each solution offers:
- Templating - scaffolding out HTML markup based on page data
- Routing - converting a request into a response
- Middleware Pipeline - building assets
- Model Binding - building usable model objects from HTML form data
- API Services - handling data requests on a server
- Client Side Interaction - updating a web page based on user interaction
For the most part, both of these frameworks offer ways to handle most of these concerns:
Feature | ASP.NET | React |
---|---|---|
Templating | ✔ | ✔ |
Routing | ✔ | ✔ |
Middleware Pipeline | ✔ | |
Model Binding | ✔ | ✔ |
API Services | ✔ | |
Client Side Interaction | ✔ |
Challenges when combining these two technologies include finding which pieces work best together.
Historically, ASP.NET has struggled to offer any sort of rich client experience out of the box. Because ASP.NET performs templating on the server, it's difficult to immediately respond to changes in state on the client without making a round trip, or writing the client side logic entirely separate from the rest of your application.
Also, React doesn't have anything to say about server-side considerations or API calls, so part of building any React app will involve picking how you want to handle requests on the server.
Here's roughly how we'll structure our architecture, with React taking care of all the client side concerns, and .NET handling the server side API.
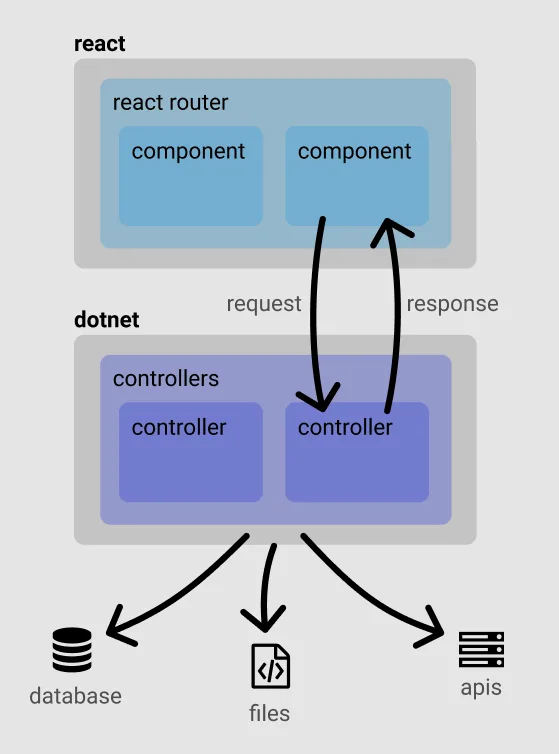
Aside: Blazor is adding rich interactivity to .NET with a monolithic API. If you want to give your existing
.cshtml
super powers, that's another possible path to pursue, but there's already a large and vibrant ecosystem for using React to take care of front end architecture.
Prerequisites - If you don't have these, you'll need em'
- Install dotnet core
- Install node & npm
- Install vs code
Getting Started
.NET comes with its own react starter template which is documented here. We'll use it for this example. You can spin up a new project with the dotnet new command like this:
dotnet new react my-new-app
Note: If you have Visual Studio, you'll also see the available template starters under the the new project dialog
You should be able to cd
into your application directory, open with VS Code, and launch with F5, or by running the following:
dotnet run
Which will be available at https://localhost:5001/
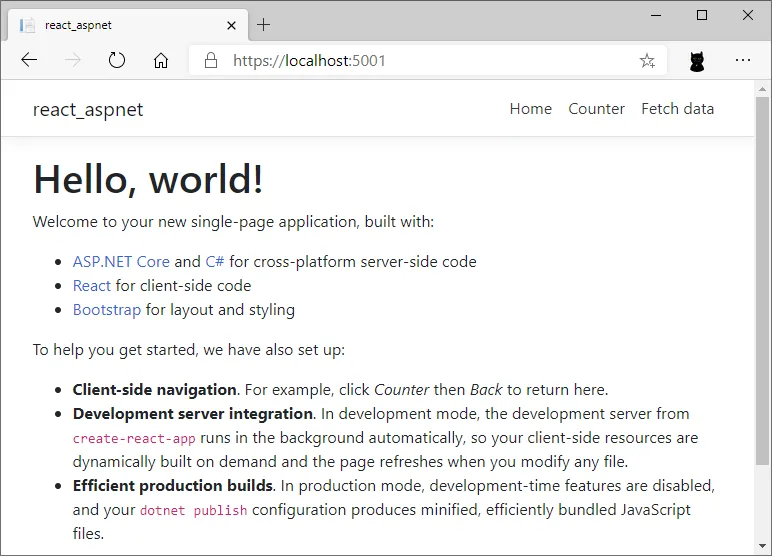
Net React Starter
Let's review what this template adds, and how all the pieces fit together
File Structure
Here's a very high level view of some of the files involved
my-app
├── .vscode/ # vs code configs
├── bin/ # generated dotnet output
├── ClientApp # React App - seeded with CRA
│ ├── build/ # generated react output
│ ├── public/ # static assets
│ ├── src/ # react app source
│ └── package.json # npm configuration
├── Controllers/ # dotnet controllers
├── Models/ # dotnet models
├── Pages/ # razor pages
├── Program.cs # dotnet entry point
├── Startup.cs # dotnet app configuration
└── my-app.csproj # project config and build steps
The startup.cs
file will wire up the react application as asp.net middleware with the following services / configuration:
// ConfigureServices()
services.AddSpaStaticFiles(configuration =>
{
configuration.RootPath = "ClientApp/build";
});
// Configure()
app.UseStaticFiles();
app.UseSpaStaticFiles();
app.UseSpa(spa =>
{
spa.Options.SourcePath = "ClientApp";
if (env.IsDevelopment())
{
spa.UseReactDevelopmentServer(npmScript: "start");
}
});
This takes advantage of the Microsoft.AspNetCore.SpaServices.Extensions
library on nuget to wire up some of the client side dependencies, and server kick off.
Finally, to wire up React to our .NET build step, there are a couple transforms in the .csproj
file which run the local build process via npm and copy the build output to the .NET project.
<Target Name="PublishRunWebpack" AfterTargets="ComputeFilesToPublish">
<!-- As part of publishing, ensure the JS resources are freshly built in production mode -->
<Exec WorkingDirectory="$(SpaRoot)" Command="npm install" />
<Exec WorkingDirectory="$(SpaRoot)" Command="npm run build" />
<!-- ... -->
</Target>
Adding Functionality - More Cats Please
And finally, let's review how to leverage all of these existing components to start adding functionality to our application. We can take advantage of the fact that API calls can happen on the server-side, allowing us to protect API Keys and tokens, while also benefiting from immediate client updates without a full page refresh.
As an example, we'll use TheCatApi, which has a quick, easy, and free sign up process to get your own API key.
For this demo project, we'll add a random image viewer, a listing of all breeds, and a navigation component using react-router to navigate between them.
We can scaffold C# classes from the sample output, and processing, through JSON2CSharp, and we'll add them to the models folder.
MVC Controller - That's the C in MVC!
The controller will orchestrate all of the backend services. Here's an example that helps setup a wrapper around the cat API so we can simplify our client side logic, and forward the request on the backend, but we could also hit a database, filesystem, or any other backend interfaces. We'll deserialize the API into a strongly typed class using the new System.Text.Json.JsonSerializer
. This is baked into .NET core, and saves us the historical dependency on Newtonsoft's JSON.NET. The API Controller can then just return data (wrapped up in a Task
because we made our method async
).
[ApiController]
[Route("api/[controller]")]
public class BreedsController : ControllerBase
[HttpGet]
public async Task<IEnumerable<Breed>> Get()
{
var BASE_URL = "https://api.thecatapi.com";
using var client = new HttpClient();
client.DefaultRequestHeaders.Add("x-api-key", _configuration["CatApiKey"]);
var resp = await client.GetStringAsync(BASE_URL + "/v1/breeds");
var breeds = JsonSerializer.Deserialize<Breed[]>(resp);
return breeds;
}
}
If we run the project, we should now be able to fetch data by navigating to /api/breed
in the browser.
You can test check if it works by itself by going to https://localhost:5001/api/breed
Now let's load that up into a React component.
React Component - It's Reactive :D
Here's the basic structure of a React component:
import React, { useState } from 'react';
export function Breeds() {
const [loading, setLoading] = useState(true);
const [breeds, setBreeds] = useState([]);
const contents = loading
? <p><em>Loading...</em></p>
: renderBreedsTable(breeds);
return (
<div>
<h1 id="tableLabel" >Get Breeds</h1>
<p>This component demonstrates fetching data from the server.</p>
{contents}
</div>
);
}
If this JavaScript is looking unfamiliar, it's because it's using JSX as well as ES6 syntax that's not supported by all browsers, which is why React needs to get preprocessed, by Babel, into something more universally understood to browsers. But let's take a look at what's going on piece-by-piece.
In order for JSX to be processed, we need to import React into our file (until React 17 that is).
The component function's primary responsibility is to return the HTML markup that we want to use one the page. Everything else is there to help manage state, or inform how to template and transform the data into HTML. So if we want a conditional, we can use the ternary operator (bool ? a : b
), or if we want to transform items in an array, we could use Array.map
to produce the output.
In order to manage state inside of a component, we use the React Hooks function useState
, which returns two items in an array that we can deconstruct like this:
const [loading, setLoading] = useState(true);
This allows us to update the state of this property and informs React to dynamically re-render the output when we do so. For example, while loading, we can display some boilerplate text until the data has returned, and then update the view appropriately.
We'll want to fetch data on load, but sending async fetch calls during the initial execution can have some weird side effects, so we can use another hook called useEffect
. Every time the component renders, we want to introduce an effect, so we'll put the initial call for data inside of there and it will fire as soon as the component goes live:
useEffect(async () => {
const response = await fetch('/api/breeds');
const data = await response.json();
setBreeds(data)
setLoading(false);
}, []);
This hits our backend API, gets the data, and updates our component state not only with the data, but also by toggling the loading indicator. Any updates to state that are used in the render function will cause it to kick off again and update the DOM automatically.
Navigation - How to get around
Let's imagine we create a second component, roughly the same as the first, to go get a random cat image. How do we handle navigation between both components. Many of the advantages to choosing React occur from preventing full page reloads on navigation. After all, your header, footer, loaded JS, and CSS libraries haven't changed - why should they get shipped to and parsed on the client from scratch. So we'll handle all navigational concerns within React as well.
Here's a pared down version of how that occurs:
- Our
index.js
file wraps the entire application inBrowserRouter
fromreact-router-dom
. - It then loads our application component which passes
Route
objects with a path and component into the overallLayout
. - The layout then renders the "live" route by injecting
this.props.children
into the rest of the site layout. - React router will handle the rest by only loading components when the path is navigated to in the browser or from other areas in the app.
// index.js
ReactDOM.render(
<BrowserRouter basename={baseUrl}>
<App />
</BrowserRouter>,
rootElement);
// app.js
export default function App() {
return (
<Layout>
<Route exact path='/' component={RandomCat} />
<Route path='/breeds' component={Breeds} />
</Layout>
);
}
// layout.js
export function Layout(props) {
return (
<div>
<NavMenu />
<Container>
{props.children}
</Container>
</div>
);
}
Wrap Up & Source Code
That's a quick run down of how you can combine both of these two technologies, and leverage the good parts of each.
There are many ways to explore each of these technologies on their own, and also try out use cases for using them together:
- If you're new to React, the current React template uses the very popular Create React App.
- If you're new to .NET, microsoft has some fantastic docs on dotnet core as well.
For more context, you can browse the full source code for the article on my Github at KyleMit/aspnet-react-cats.
What would you like to see added to this example next? Tweet me at KyleMitBTV.