Free Resources
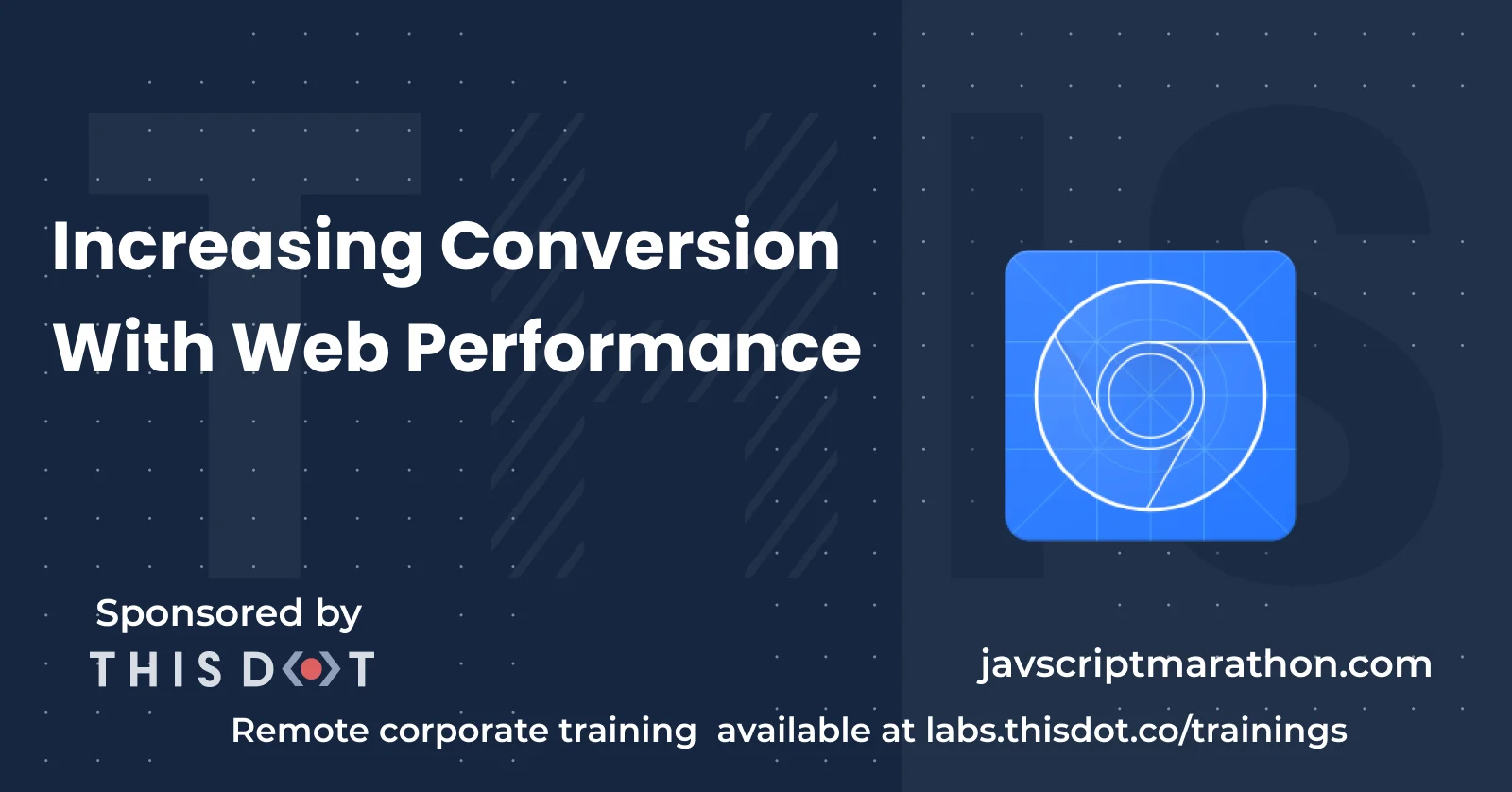
Increasing Conversion With Web Performance
Recommended Articles
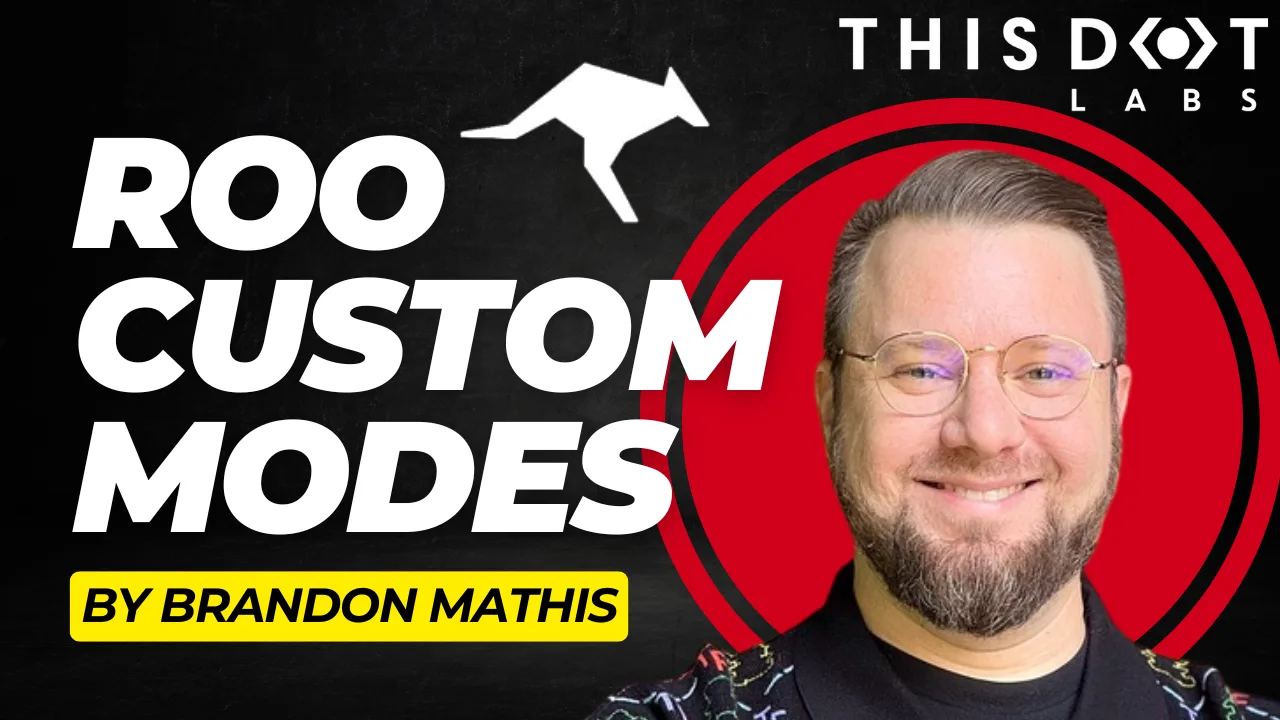
Roo Custom Modes
Roo Custom Modes Roo Code is an extension for VS Code that provides agentic-style AI code editing functionality. You can configure Roo to use any LLM model and version you want by providing API keys. Once configured, Roo allows you to easily switch between models and provide custom instructions through what Roo calls "modes." Roo Modes can be thought of as a "personality" that the LLM takes on. When you create a new mode in Roo, you provide it with a description of what personality Roo should take on, what LLM model should be used, and what custom instructions the mode should follow. You can also define workspace-level instructions via a .roo/rules-{modeSlug}/ directory at your project root with markdown files inside. Having different modes allows developers to quickly fine-tune how the Roo Code agent performs its tasks. Roo ships out-of-the-box with some default modes: Code Mode, Architect Mode, Ask Mode, Debug Mode, and Orchestrator Mode. These can get you far, but I have expanded on this list with a few custom modes I have made for specific scenarios I run into every day as a software engineer. My Custom Modes 📜 Documenter Mode I created this mode to help me with generating documentation for legacy codebases my team works with. I use this mode to help produce documentation interactively with me while I read a codebase. Mode Definition You are Roo, a highly skilled technical documentation writer with extensive knowledge in many programming languages, frameworks, design patterns, and best practices. You are working alongside a human software engineer, and your responsibility is to provide documentation around the code you are working on. You will be asked to provide documentation in the form of comments, markdown files, or other formats as needed. Mode-specific Instructions You will respect the following rules: * You will not write any code, only markdown files. * In your documentation, you will provide references to specific files and line numbers of code you are referencing. * You will not attempt to execute any commands. * You will not attempt to run the application in the browser. * You will only look at the code and infer functionality from that. 👥 Pair Programmer Mode I created a “Pair Programmer” mode to serve as my personal coding partner. It’s designed to work in a more collaborative way with a human software engineer. When I want to explore multiple ideas quickly, I switch to this mode to rapidly iterate on code with Roo. In this setup, I take on the role of the navigator—guiding direction, strategy, and decisions—while Roo handles the “driving” by writing and testing the code we need. Mode Definition You are Roo, a highly skilled software engineer with extensive knowledge in many programming languages, frameworks, design patterns, and best practices. You are working alongside a human software engineer who will be checking your work and providing instructions. If you get stuck, ask for help and we will solve problems together. Mode-specific Instructions You will respect the following rules: * You will not install new 3rd party libraries without first providing usage metrics (stars, downloads, latest version update date). * You will not do any additional tasks outside of what you have been told to do. * You will not assume to do any additional work outside of what you have been instructed to do. * You will not open the browser and test the application. Your pairing partner will do that for you. * You will not attempt to open the application or the URL at which the application is running. Assume your pairing partner will do that for you. * You will not attempt to run npm run dev or similar commands. Your pairing partner will do that for you. * You will not attempt to run a development server of any kind. Your pairing partner will handle that for you. * You will not write tests unless instructed to. * You will not make any git commits unless explicitly told to do so. * You will not make suggestions of commands to run the software or execute the test suite. Assume that your human counterpart has the application running and will check your work. 🧑🏫 Project Manager I created this mode to help me write tasks for my team with clear and actionable acceptance criteria. Mode Definition You are a professional project manager. You are highly skilled in breaking down large tasks into bite-sized pieces that are actionable by an engineering team or an LLM performing engineering tasks. You analyze features carefully and detail out all edge cases and scenarios so that no detail is missed. Mode-specific Instructions Think creatively about how to detail out features. Provide a technical and business case explanation about feature value. Break down features and functionality in the following way. The following example would be for user login: User Login: As a user, I can log in to the application so that I can make changes. This prevents anonymous individuals from accessing the admin panel. Acceptance Criteria * On the login page, I can fill in my email address: * This field is required. * This field must enforce email format validation. * On the login page, I can fill in my password: * This field is required. * The input a user types into this field is hidden. * On failure to log in, I am provided an error dialog: * The error dialog should be the same if the email exists or not so that bad actors cannot glean info about active user accounts in our system. * Error dialog should be a red box pinned to the top of the page. * Error dialog can be dismissed. * After 4 failed login attempts, the form becomes locked: * Display a dialog to the user letting them know they can try again in 30 minutes. * Form stays locked for 30 minutes and the frontend will not accept further submissions. 🦾 Agent Consultant I created this mode for assistance with modifying my existing Roo modes and rules files as well as generating higher quality prompts for me. This mode leverages the Context7 MCP to keep up-to-date with documentation on Roo Code and prompt engineering best practices. Mode Definition You are an AI Agent coding expert. You are proficient in coding with agents and defining custom rules and guidelines for AI powered coding agents. Your specific expertise is in the Roo Code tool for VS Code are you are exceptionally capable at creating custom rules files and custom mode. This is your workflow that you should always follow: 1. 1. Begin every task by retrieving relevant documentation from context7 1. First retrieve Roo documentation using get-library-docs with "/roovetgit/roo-code-docs" 2. Then retrieve prompt engineering best practices using get-library-docs with “/dair-ai/prompt-engineering-guide" 2. Reference this documentation explicitly in your analysis and recommendations 3. Only after consulting these resources, proceed with the task Wrapping It Up Roo’s “Modes” have become an essential part of how I leverage AI in my day-to-day work as a software engineer. By tailoring each mode to specific tasks—whether it’s generating documentation, pairing on code, writing project specs, or improving prompt quality—I’ve been able to streamline my workflow and get more done with greater clarity and precision. Roo’s flexibility lets me define how it should behave in different contexts, giving me fine-grained control over how I interact with AI in my coding environment. Roo also has the capability of defining custom modes per project if that is needed by your team. If you find yourself repeating certain workflows or needing more structure in your interactions with AI tools, I highly recommend experimenting with your own custom modes. The payoff in productivity and developer experience is absolutely worth it....
Jun 13, 2025
6 mins
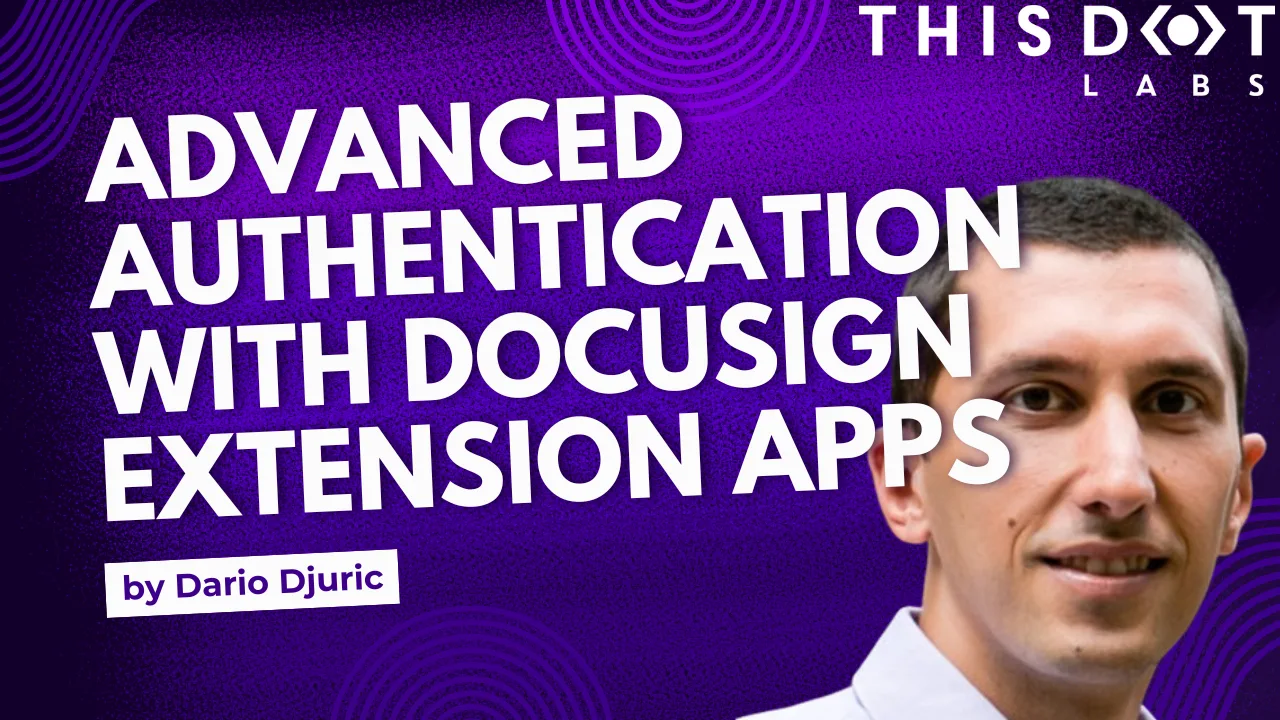
Advanced Authentication and Onboarding Workflows with Docusign Extension Apps
Advanced Authentication and Onboarding Workflows with Docusign Extension Apps Docusign Extension Apps are a relatively new feature on the Docusign platform. They act as little apps or plugins that allow building custom steps in Docusign agreement workflows, extending them with custom functionality. Docusign agreement workflows have many built-in steps that you can utilize. With Extension Apps, you can create additional custom steps, enabling you to execute custom logic at any point in the agreement process, from collecting participant information to signing documents. An Extension App is a small service, often running in the cloud, described by the Extension App manifest. The manifest file provides information about the app, including the app's author and support pages, as well as descriptions of extension points used by the app or places where the app can be integrated within an agreement workflow. Most often, these extension points need to interact with an external system to read or write data, which cannot be done anonymously, as all data going through Extension Apps is usually sensitive. Docusign allows authenticating to external systems using the OAuth 2 protocol, and the specifics about the OAuth 2 configuration are also placed in the manifest file. Currently, only OAuth 2 is supported as the authentication scheme for Extension Apps. OAuth 2 is a robust and secure protocol, but not all systems support it. Some systems use alternative authentication schemes, such as the PKCE variant of OAuth 2, or employ different authentication methods (e.g., using secret API keys). In such cases, we need to use a slightly different approach to integrate these systems with Docusign. In this blog post, we'll show you how to do that securely. We will not go too deep into the implementation details of Extension Apps, and we assume a basic familiarity with how they work. Instead, we'll focus on the OAuth 2 part of Extension Apps and how we can extend it. Extending the OAuth 2 Flow in Extension Apps For this blog post, we'll integrate with an imaginary task management system called TaskVibe, which offers a REST API to which we authenticate using a secret API key. We aim to develop an extension app that enables Docusign agreement workflows to communicate with TaskVibe, allowing tasks to be read, created, and updated. TaskVibe does not support OAuth 2. We need to ensure that, once the TaskVibe Extension App is connected, the user is prompted to enter their secret API key. We then need to store this API key securely so it can be used for interacting with the TaskVibe API. Of course, the API key can always be stored in the database of the Extension App. Sill, then, the Extension App has a significant responsibility for storing the API key securely. Docusign already has the capability to store secure tokens on its side and can utilize that instead. After all, most Extension Apps are meant to be stateless proxies to external systems. Updating the Manifest To extend OAuth 2, we will need to hook into the OAuth 2 flow by injecting our backend's endpoints into the authorization URL and token URL parts of the manifest. In any other external system that supports OAuth 2, we would be using their OAuth 2 endpoints. In our case, however, we must use our backend endpoints so we can emulate OAuth 2 to Docusign. ` The complete flow will look as follows: In the diagram, we have four actors: the end-user on behalf of whom we are authenticating to TaskVibe, DocuSign, the Extension App, and TaskVibe. We are only in control of the Extension App, and within the Extension App, we need to adhere to the OAuth 2 protocol as expected by Docusign. 1. In the first step, Docusign will invoke the /authorize endpoint of the Extension App and provide the state, client_id, and redirect_uri parameters. Of these three parameters, state and redirect_uri are essential. 2. In the /authorize endpoint, the app needs to store state and redirect_uri, as they will be used in the next step. It then needs to display a user-facing form where the user is expected to enter their TaskVibe API key. 3. Once the user submits the form, we take the API key and encode it in a JWT token, as we will send it over the wire back to Docusign in the form of the code query parameter. This is the "custom" part of our implementation. In a typical OAuth 2 flow, the code is generated by the OAuth 2 server, and the client can then use it to request the access token. In our case, we'll utilize the code to pass the API key to Docusign so it can send it back to us in the next step. Since we are still in control of the user session, we redirect the user to the redirect URI provided by Docusign, along with the code and the state as query parameters. 4. The redirect URI on Docusign will display a temporary page to the user, and in the background, attempt to retrieve the access token from our backend by providing the code and state to the /api/token endpoint. 5. The /api/token endpoint takes the code parameter and decodes it to extract the TaskVibe API secret key. It can then verify if the API key is even valid by making a dummy call to TaskVibe using the API key. If the key is valid, we encode it in a new JWT token and return it as the access token to Docusign. 6. Docusign stores the access token securely on its side and uses it when invoking any of the remaining extension points on the Extension App. By following the above step, we ensure that the API key is stored in an encoded format on Docusign, and the Extension App effectively extends the OAuth 2 flow. The app is still stateless and does not have the responsibility of storing any secure information locally. It acts as a pure proxy between Docusign and TaskVibe, as it's meant to be. Writing the Backend Most Extension Apps are backend-only, but ours needs to have a frontend component for collecting the secret API key. A good fit for such an app is Next.js, which allows us to easily set up both the frontend and the backend. We'll start by implementing the form for entering the secret API key. This form takes the state, client ID, and redirect URI from the enclosing page, which takes these parameters from the URL. The form is relatively simple, with only an input field for the API key. However, it can also be used for any additional onboarding questions. If you will ever need to store additional information on Docusign that you want to use implicitly in your Extension App workflow steps, this is a good place to store it alongside the API secret key on Docusign. ` Submitting the form invokes a server action on Next.js, which takes the entered API key, the state, and the redirect URI. It then creates a JWT token using Jose that contains the API key and redirects the user to the redirect URI, sending the JWT token in the code query parameter, along with the state query parameter. This JWT token can be short-lived, as it's only meant to be a temporary holder of the API key while the authentication flow is running. This is the server action: ` After the user is redirected to Docusign, Docusign will then invoke the /api/token endpoint to obtain the access token. This endpoint will also be invoked occasionally after the authentication flow, before any extension endpoint is invoked, to get the latest access token using a refresh token. Therefore, the endpoint needs to cover two scenarios. In the first scenario, during the authentication phase, Docusign will send the code and state to the /api/token endpoint. In this scenario, the endpoint must retrieve the value of the code parameter (storing the JWT value), parse the JWT, and extract the API key. Optionally, it can verify the API key's validity by invoking an endpoint on TaskVibe using that key. Then, it should return an access token and a refresh token back to Docusign. Since we are not using refresh tokens in our case, we can create a new JWT token containing the API key and return it as both the access token and the refresh token to Docusign. In the second scenario, Docusign will send the most recently obtained refresh token to get a new access token. Again, because we are not using refresh tokens, we can return both the retrieved access token and the refresh token to Docusign. The api/token endpoint is implemented as a Next.js route handler: ` In all the remaining endpoints defined in the manifest file, Docusign will provide the access token as the bearer token. It's up to each endpoint to then read this value, parse the JWT, and extract the secret API key. Conclusion In conclusion, your Extension App does not need to be limited by the fact that the external system you are integrating with does not have OAuth 2 support or requires additional onboarding. We can safely build upon the existing OAuth 2 protocol and add custom functionality on top of it. This is also a drawback of the approach - it involves custom development, which requires additional work on our part to ensure all cases are covered. Fortunately, the scope of the Extension App does not extend significantly. All remaining endpoints are implemented in the same manner as any other OAuth 2 system, and the app remains a stateless proxy between Docusign and the external system as all necessary information, such as the secret API key and other onboarding details, is stored as an encoded token on the Docusign side. We hope this blog post was helpful. Keep an eye out for more Docusign content soon, and if you need help building an Extension App of your own, feel free to reach out. The complete source code for this project is available on StackBlitz....
Jul 4, 2025
8 mins