Free Resources
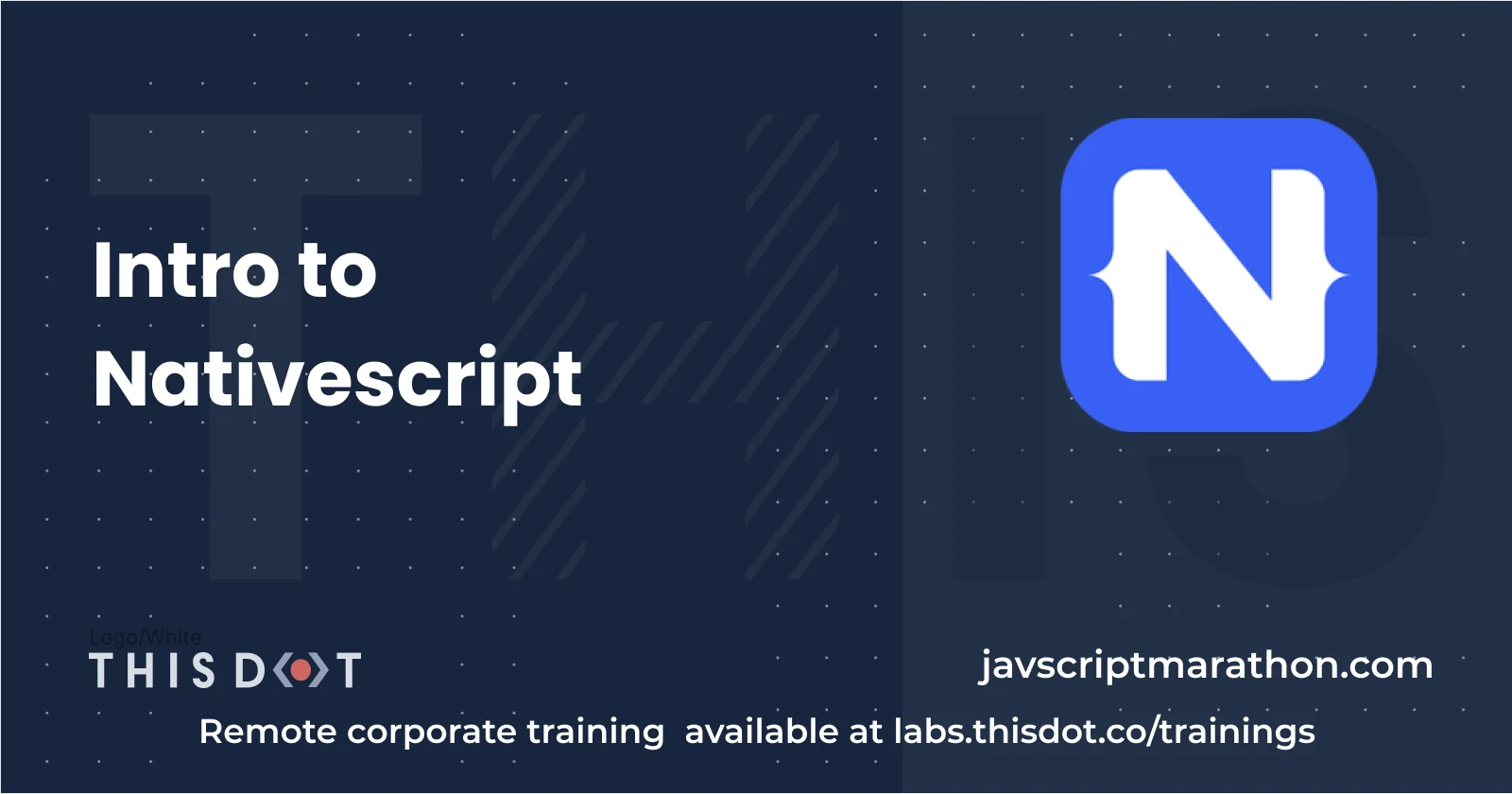
Intro to NativeScript
Recommended Articles
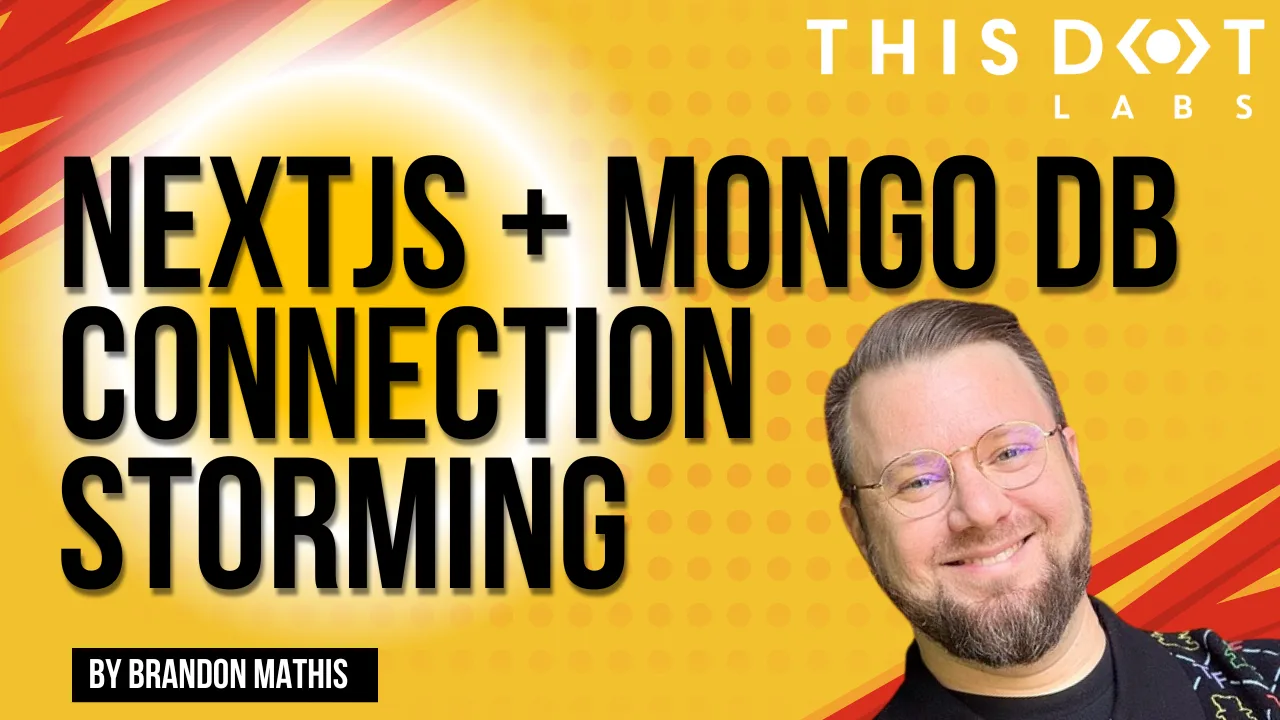
Next.js + MongoDB Connection Storming
Building a Next.js application connected to MongoDB can feel like a match made in heaven. MongoDB stores all of its data as JSON objects, which don’t require transformation into JavaScript objects like relational SQL data does. However, when deploying your application to a serverless production environment such as Vercel, it is crucial to manage your database connections properly. If you encounter errors like these, you may be experiencing Connection Storming: * MongoServerSelectionError: connect ECONNREFUSED <IP_ADDRESS>:<PORT> * MongoNetworkError: failed to connect to server [<hostname>:<port>] on first connect * MongoTimeoutError: Server selection timed out after <x> ms * MongoTopologyClosedError: Topology is closed, please connect * Mongo Atlas: Connections % of configured limit has gone above 80 Connection storming occurs when your application has to mount a connection to Mongo for every serverless function or API endpoint call. Vercel executes your application’s code in a highly concurrent and isolated fashion. So, if you create new database connections on each request, your app might quickly exceed the connection limit of your database. We can leverage Vercel’s fluid compute model to keep our database connection objects warm across function invocations. Traditional serverless architecture was designed for quick, stateless web app transactions. Now, especially with the rise of LLM-oriented applications built with Next.js, interactions with applications are becoming more sequential. We just need to ensure that we assign our MongoDB connection to a global variable. Protip: Use global variables Vercel’s fluid compute model means all memory, including global constants like a MongoDB client, stays initialized between requests as long as the instance remains active. By assigning your MongoDB client to a global constant, you avoid redundant setup work and reduce the overhead of cold starts. This enables a more efficient approach to reusing connections for your application’s MongoDB client. The example below demonstrates how to retrieve an array of users from the users collection in MongoDB and either return them through an API request to /api/users or render them as an HTML list at the /users route. To support this, we initialize a global clientPromise variable that maintains the MongoDB connection across warm serverless executions, avoiding re-initialization on every request. ` Using this database connection in your API route code is easy: ` You can also use this database connection in your server-side rendered React components. ` In serverless environments like Vercel, managing database connections efficiently is key to avoiding connection storming. By reusing global variables and understanding the serverless execution model, you can ensure your Next.js app remains stable and performant....
Jul 11, 2025
3 mins
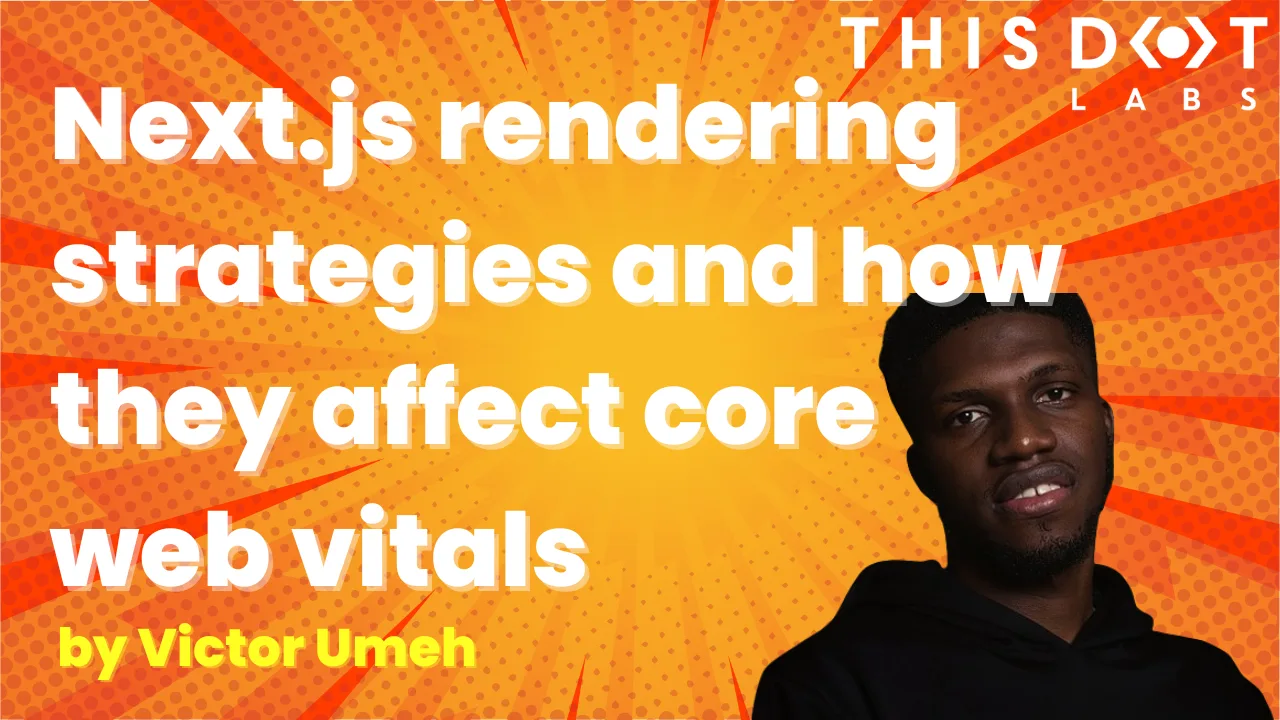
Next.js Rendering Strategies and how they affect core web vitals
When it comes to building fast and scalable web apps with Next.js, it’s important to understand how rendering works, especially with the App Router. Next.js organizes rendering around two main environments: the server and the client. On the server side, you’ll encounter three key strategies: Static Rendering, Dynamic Rendering, and Streaming. Each one comes with its own set of trade-offs and performance benefits, so knowing when to use which is crucial for delivering a great user experience. In this post, we'll break down each strategy, what it's good for, and how it impacts your site's performance, especially Core Web Vitals. We'll also explore hybrid approaches and provide practical guidance on choosing the right strategy for your use case. What Are Core Web Vitals? Core Web Vitals are a set of metrics defined by Google that measure real-world user experience on websites. These metrics play a major role in search engine rankings and directly affect how users perceive the speed and smoothness of your site. * Largest Contentful Paint (LCP): This measures loading performance. It calculates the time taken for the largest visible content element to render. A good LCP is 2.5 seconds or less. * Interaction to Next Paint (INP): This measures responsiveness to user input. A good INP is 200 milliseconds or less. * Cumulative Layout Shift (CLS): This measures the visual stability of the page. It quantifies layout instability during load. A good CLS is 0.1 or less. If you want to dive deeper into Core Web Vitals and understand more about their impact on your website's performance, I recommend reading this detailed guide on New Core Web Vitals and How They Work. Next.js Rendering Strategies and Core Web Vitals Let's explore each rendering strategy in detail: 1. Static Rendering (Server Rendering Strategy) Static Rendering is the default for Server Components in Next.js. With this approach, components are rendered at build time (or during revalidation), and the resulting HTML is reused for each request. This pre-rendering happens on the server, not in the user's browser. Static rendering is ideal for routes where the data is not personalized to the user, and this makes it suitable for: * Content-focused websites: Blogs, documentation, marketing pages * E-commerce product listings: When product details don't change frequently * SEO-critical pages: When search engine visibility is a priority * High-traffic pages: When you want to minimize server load How Static Rendering Affects Core Web Vitals * Largest Contentful Paint (LCP): Static rendering typically leads to excellent LCP scores (typically < 1s). The Pre-rendered HTML can be cached and delivered instantly from CDNs, resulting in very fast delivery of the initial content, including the largest element. Also, there is no waiting for data fetching or rendering on the client. * Interaction to Next Paint (INP): Static rendering provides a good foundation for INP, but doesn't guarantee optimal performance (typically ranges from 50-150 ms depending on implementation). While Server Components don't require hydration, any Client Components within the page still need JavaScript to become interactive. To achieve a very good INP score, you will need to make sure the Client Components within the page is minimal. * Cumulative Layout Shift (CLS): While static rendering delivers the complete page structure upfront which can be very beneficial for CLS, achieving excellent CLS requires additional optimization strategies: * Static HTML alone doesn't prevent layout shifts if resources load asynchronously * Image dimensions must be properly specified to reserve space before the image loads * Web fonts can cause text to reflow if not handled properly with font display strategies * Dynamically injected content (ads, embeds, lazy-loaded elements) can disrupt layout stability * CSS implementation significantly impacts CLS—immediate availability of styling information helps maintain visual stability Code Examples: 1. Basic static rendering: ` 2. Static rendering with revalidation (ISR): ` 3. Static path generation: ` 2. Dynamic Rendering (Server Rendering Strategy) Dynamic Rendering generates HTML on the server for each request at request time. Unlike static rendering, the content is not pre-rendered or cached but freshly generated for each user. This kind of rendering works best for: * Personalized content: User dashboards, account pages * Real-time data: Stock prices, live sports scores * Request-specific information: Pages that use cookies, headers, or search parameters * Frequently changing data: Content that needs to be up-to-date on every request How Dynamic Rendering Affects Core Web Vitals * Largest Contentful Paint (LCP): With dynamic rendering, the server needs to generate HTML for each request, and that can't be fully cached at the CDN level. It is still faster than client-side rendering as HTML is generated on the server. * Interaction to Next Paint (INP): The performance is similar to static rendering once the page is loaded. However, it can become slower if the dynamic content includes many Client Components. * Cumulative Layout Shift (CLS): Dynamic rendering can potentially introduce CLS if the data fetched at request time significantly alters the layout of the page compared to a static structure. However, if the layout is stable and the dynamic content size fits within predefined areas, the CLS can be managed effectively. Code Examples: 1. Explicit dynamic rendering: ` 2. Simplicit dynamic rendering with cookies: ` 3. Dynamic routes: ` 3. Streaming (Server Rendering Strategy) Streaming allows you to progressively render UI from the server. Instead of waiting for all the data to be ready before sending any HTML, the server sends chunks of HTML as they become available. This is implemented using React's Suspense boundary. React Suspense works by creating boundaries in your component tree that can "suspend" rendering while waiting for asynchronous operations. When a component inside a Suspense boundary throws a promise (which happens automatically with data fetching in React Server Components), React pauses rendering of that component and its children, renders the fallback UI specified in the Suspense component, continues rendering other parts of the page outside this boundary, and eventually resumes and replaces the fallback with the actual component once the promise resolves. When streaming, this mechanism allows the server to send the initial HTML with fallbacks for suspended components while continuing to process suspended components in the background. The server then streams additional HTML chunks as each suspended component resolves, including instructions for the browser to seamlessly replace fallbacks with final content. It works well for: * Pages with mixed data requirements: Some fast, some slow data sources * Improving perceived performance: Show users something quickly while slower parts load * Complex dashboards: Different widgets have different loading times * Handling slow APIs: Prevent slow third-party services from blocking the entire page How Streaming Affects Core Web Vitals * Largest Contentful Paint (LCP): Streaming can improve the perceived LCP. By sending the initial HTML content quickly, including potentially the largest element, the browser can render it sooner. Even if other parts of the page are still loading, the user sees the main content faster. * Interaction to Next Paint (INP): Streaming can contribute to a better INP. When used with React's <Suspense />, interactive elements in the faster-loading parts of the page can become interactive earlier, even while other components are still being streamed in. This allows users to engage with the page sooner. * Cumulative Layout Shift (CLS): Streaming can cause layout shifts as new content streams in. However, when implemented carefully, streaming should not negatively impact CLS. The initially streamed content should establish the main layout, and subsequent streamed chunks should ideally fit within this structure without causing significant reflows or layout shifts. Using placeholders and ensuring dimensions are known can help prevent CLS. Code Examples: 1. Basic Streaming with Suspense: ` 2. Nested Suspense boundaries for more granular control: ` 3. Using Next.js loading.js convention: ` 4. Client Components and Client-Side Rendering Client Components are defined using the React 'use client' directive. They are pre-rendered on the server but then hydrated on the client, enabling interactivity. This is different from pure client-side rendering (CSR), where rendering happens entirely in the browser. In the traditional sense of CSR (where the initial HTML is minimal, and all rendering happens in the browser), Next.js has moved away from this as a default approach but it can still be achievable by using dynamic imports and setting ssr: false. ` Despite the shift toward server rendering, there are valid use cases for CSR: 1. Private dashboards: Where SEO doesn't matter, and you want to reduce server load 2. Heavy interactive applications: Like data visualization tools or complex editors 3. Browser-only APIs: When you need access to browser-specific features like localStorage or WebGL 4. Third-party integrations: Some third-party widgets or libraries that only work in the browser While these are valid use cases, using Client Components is generally preferable to pure CSR in Next.js. Client Components give you the best of both worlds: server-rendered HTML for the initial load (improving SEO and LCP) with client-side interactivity after hydration. Pure CSR should be reserved for specific scenarios where server rendering is impossible or counterproductive. Client components are good for: * Interactive UI elements: Forms, dropdowns, modals, tabs * State-dependent UI: Components that change based on client state * Browser API access: Components that need localStorage, geolocation, etc. * Event-driven interactions: Click handlers, form submissions, animations * Real-time updates: Chat interfaces, live notifications How Client Components Affect Core Web Vitals * Largest Contentful Paint (LCP): Initial HTML includes the server-rendered version of Client Components, so LCP is reasonably fast. Hydration can delay interactivity but doesn't necessarily affect LCP. * Interaction to Next Paint (INP): For Client Components, hydration can cause input delay during page load, and when the page is hydrated, performance depends on the efficiency of event handlers. Also, complex state management can impact responsiveness. * Cumulative Layout Shift (CLS): Client-side data fetching can cause layout shifts as new data arrives. Also, state changes might alter the layout unexpectedly. Using Client Components will require careful implementation to prevent shifts. Code Examples: 1. Basic Client Component: ` 2. Client Component with server data: ` Hybrid Approaches and Composition Patterns In real-world applications, you'll often use a combination of rendering strategies to achieve the best performance. Next.js makes it easy to compose Server and Client Components together. Server Components with Islands of Interactivity One of the most effective patterns is to use Server Components for the majority of your UI and add Client Components only where interactivity is needed. This approach: 1. Minimizes JavaScript sent to the client 2. Provides excellent initial load performance 3. Maintains good interactivity where needed ` Partial Prerendering (Next.js 15) Next.js 15 introduced Partial Prerendering, a new hybrid rendering strategy that combines static and dynamic content in a single route. This allows you to: 1. Statically generate a shell of the page 2. Stream in dynamic, personalized content 3. Get the best of both static and dynamic rendering Note: At the time of this writing, Partial Prerendering is experimental and is not ready for production use. Read more ` Measuring Core Web Vitals in Next.js Understanding the impact of your rendering strategy choices requires measuring Core Web Vitals in real-world conditions. Here are some approaches: 1. Vercel Analytics If you deploy on Vercel, you can use Vercel Analytics to automatically track Core Web Vitals for your production site: ` 2. Web Vitals API You can manually track Core Web Vitals using the web-vitals library: ` 3. Lighthouse and PageSpeed Insights For development and testing, use: * Chrome DevTools Lighthouse tab * PageSpeed Insights * Chrome User Experience Report Making Practical Decisions: Which Rendering Strategy to Choose? Choosing the right rendering strategy depends on your specific requirements. Here's a decision framework: Choose Static Rendering when * Content is the same for all users * Data can be determined at build time * Page doesn't need frequent updates * SEO is critical * You want the best possible performance Choose Dynamic Rendering when * Content is personalized for each user * Data must be fresh on every request * You need access to request-time information * Content changes frequently Choose Streaming when * Page has a mix of fast and slow data requirements * You want to improve perceived performance * Some parts of the page depend on slow APIs * You want to prioritize showing critical UI first Choose Client Components when * UI needs to be interactive * Component relies on browser APIs * UI changes frequently based on user input * You need real-time updates Conclusion Next.js provides a powerful set of rendering strategies that allow you to optimize for both performance and user experience. By understanding how each strategy affects Core Web Vitals, you can make informed decisions about how to build your application. Remember that the best approach is often a hybrid one, combining different rendering strategies based on the specific requirements of each part of your application. Start with Server Components as your default, use Static Rendering where possible, and add Client Components only where interactivity is needed. By following these principles and measuring your Core Web Vitals, you can create Next.js applications that are fast, responsive, and provide an excellent user experience....
May 30, 2025
9 mins